Microanalytics: Enhancing Data-Driven Decisions
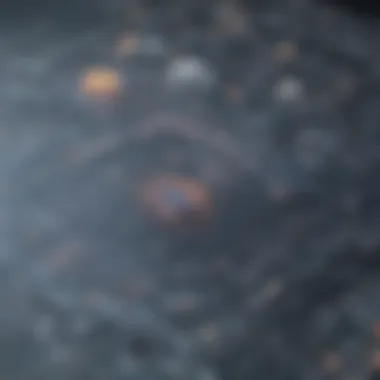
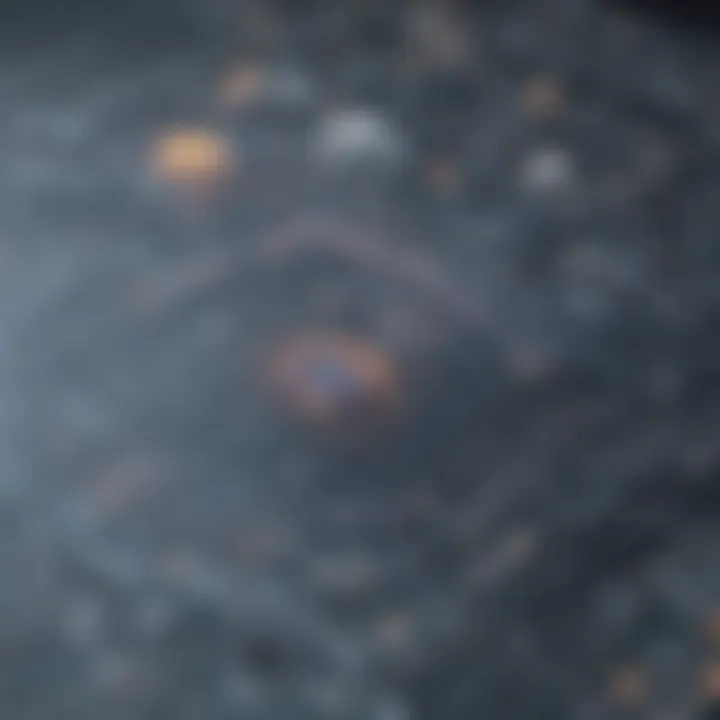
Intro
Microanalytics is rapidly becoming a pivotal element in the framework of informed decision-making within businesses. It refers to the granular analysis of data, often sourced from everyday interactions or operational processes, allowing organizations to gain rich insights. The growing emphasis on data-driven strategies has compelled leaders to adopt microanalytics, enhancing their capacity to understand nuanced trends and patterns that larger analytics might overlook.
In this article, we will delve into the features and functionalities that distinguish microanalytics as a vital tool for small to medium-sized businesses, entrepreneurs, and IT professionals. By unpacking the unique selling points and methods employed in microanalytics, readers will gain a comprehensive understanding of how it can transform decision-making processes.
Furthermore, we will examine the challenges and ethical considerations that come with implementing these tools. Each section will synthesize these elements to provide a clear view on leveraging microanalytics in a practical manner, leading to optimized business strategies.
Overview of Features
Microanalytics offers several functionalities that enhance how organizations can analyze and interpret data. This section will explore these features and their benefits.
Highlight significant functionalities
- Real-Time Data Collection: Microanalytics facilitates constant data monitoring. This means that businesses can analyze trends as they happen, adapting their strategies in response to immediate changes in the market.
- Granular Insight Generation: Unlike broader analysis that may aggregate data, microanalytics breaks down data into smaller units. This allows for the identification of specific behaviors, product usage heights, and customer preferences.
- Integration with Existing Tools: Microanalytics can often be integrated into existing software systems, such as Customer Relationship Management (CRM) or ERP platforms. This seamless integration ensures that analysts and managers can leverage microanalytics without overhauling their entire data infrastructure.
- Predictive Analysis Capabilities: With the advancement of machine learning, microanalytics can anticipate future trends based on small data patterns. This predictive element aids in proactive strategy formulation.
Explain how they benefit users
The functionalities mentioned above offer distinct advantages:
- Improved agility in decision-making as businesses can react quickly to new information.
- Enhanced understanding of customer preferences leads to more tailored marketing strategies.
- Increased operational efficiency through better resource allocation based on precise data insights.
- Informed strategic planning based on gradual trends rather than sporadic data points.
Effective use of microanalytics often results in higher ROI for marketing campaigns and streamlined operational processes.
By taking advantage of these features, organizations can drive better outcomes and achieve their strategic goals more effectively.
Unique Selling Points
What distinguishes microanalytics from conventional analytics practices? This section aims to capture its unique aspects that contribute to its increasing adoption.
What sets this framework apart from competitors
- Focus on Micro-Level Data: While many analytic frameworks focus on larger data sets, microanalytics emphasizes small-scale data specifics. This creates clarity in understanding intricate details of customer behavior and market dynamics.
- User-Centric Interface: Many microanalytics platforms emphasize ease of use. This user-friendly design encourages adoption across teams, ensuring that even non-technical members can benefit from data-driven insights.
- Scalability: Microanalytics frameworks are typically designed to grow with the organization. They can handle an increasing amount of data without compromising performance, making them a worthwhile investment.
Emphasis on innovation or specific capabilities
Innovation is at the heart of microanalytics development. Features like artificial intelligence integration and real-time analysis capabilities set it apart. This focus on cutting-edge technologies allows businesses to remain competitive.
As we continue to explore microanalytics, understanding its frameworks, implications on operational efficiency, and the challenges associated with its implementation will provide invaluable knowledge. This will ultimately guide executives and managers in optimizing their business strategies, ensuring that they are well-equipped to take advantage of microanalytics.
In this evolving landscape, the demand for precise, actionable insights is higher than ever. Microanalytics is not just another tool; it is a necessary approach for those who aim to navigate an increasingly complex business environment.
Understanding Microanalytics
Microanalytics represents a critical shift in how businesses approach data analysis and decision-making. In today’s fast-paced environment, microanalytics equips organizations with the capacity to delve into detailed, granular data rather than relying solely on broader metrics. This targeted examination allows businesses to understand their operations on a more intimate level—the "micro" perspective—driving more informed and precise decisions.
Understanding microanalytics becomes essential for executives and managers aiming to enhance their operational strategies. This framework fosters adaptability, enabling businesses to respond swiftly to emerging trends and consumer behaviors. With a detailed insight into specific datasets, organizations can optimize their processes and strategies accordingly, ultimately enhancing their competitive edge.
Definition of Microanalytics
Microanalytics refers to the practice of analyzing small units of data to extract actionable insights. It goes beyond traditional analytics by focusing on specific elements within a dataset, rather than merely assessing aggregated data. This practice often involves real-time data collection and analysis, allowing decision-makers to monitor and adjust strategies as needed. For instance, a company can investigate customer interactions on a website, analyzing the clicks and navigation patterns to optimize the user experience. This level of granularity can uncover trends that larger, traditional datasets might overlook, leading to more effective interventions.
Contrast with Traditional Analytics
While traditional analytics generally examines extensive data sets to derive conclusions, microanalytics targets specific details, providing a sharper understanding of underlying trends. Traditional approaches may incorporate statistical methods to summarize data across broad categories, such as overall sales figures of a product line. In contrast, microanalytics can focus on the behavior of individual customers and their interactions with specific products, helping identify niche preferences and even predict future buying behaviors.
The practical implications are significant:
- Timeliness: Microanalytics often allows for real-time analysis, enabling quicker decision-making.
- Precision: Organizations gain targeted insights that are often missed in traditional analyses.
- Adaptability: Microanalytics supports ongoing adjustments to strategies, informed by the latest data.
Microanalytics transforms data into a practical tool, enhancing both decision-making and operational effectiveness.
In summary, understanding microanalytics is paramount for small to medium-sized businesses, entrepreneurs, and IT professionals. This knowledge enables them to leverage detailed insights, ultimately fostering more informed, strategic decisions.
The Significance of Microanalytics
Microanalytics plays a crucial role in today's business landscape, particularly for small to medium-sized enterprises. Its significance stems from the transformative potential it has for decision-making based on data insights. Businesses must navigate evolving markets, and microanalytics provides the framework to analyze data at a granular level. This detailed analysis helps organizations gain insights that enhance strategic decisions and operations.
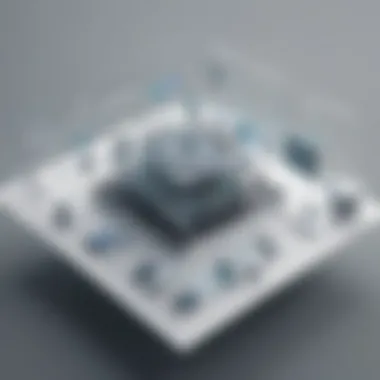
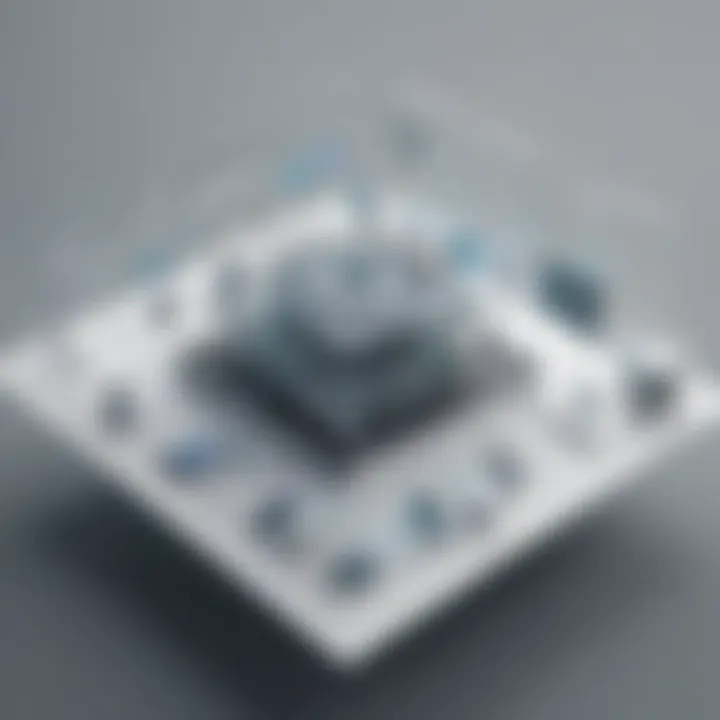
There are several key reasons why microanalytics is essential:
- Informed Decision-Making: By analyzing specific data points rather than relying on broad averages, companies can tailor their strategies.
- Action-Oriented Insights: Microanalytics allows businesses to identify actionable insights quickly, making it possible to adapt to changing circumstances.
- Customization and Personalization: Companies can understand individual customer behaviors and preferences, leading to enhanced customer satisfaction.
Microanalytics serves as a bridge that connects data to effective action. This connection is vital for agencies looking to make tactical adjustments to their operations.
Enhancing Decision-Making Processes
In the context of decision-making processes, microanalytics brings precision and clarity. Businesses can use micro-level data to inform their strategies. It goes beyond traditional analytics by providing specifics that typical measures might overlook. For instance, while traditional analytics could suggest a decline in sales in a specific region, microanalytics could reveal that a particular product is underperforming due to a local competition or a supply chain issue.
Furthermore, decision-makers can segment data into meaningful categories. By leveraging user profiling and behavior trends, businesses can make more accurate predictions about customer needs and market movements.
- Enhanced Responsiveness: Organizations can respond promptly to emerging trends. Timeliness is critical in today's fast-paced environment.
- Data-Driven Confidence: Leaders gain confidence when making decisions backed by detailed analysis rather than gut feelings.
- Improved Risk Management: Identifying troubling trends becomes easier, allowing businesses to address potential issues before they escalate.
Boosting Operational Efficiency
Microanalytics also contributes significantly to operational efficiency. It allows businesses to streamline processes by identifying bottlenecks and inefficiencies. With specific data insights, organizations can focus on optimizing relevant areas rather than making sweeping changes across the board.
For example, in a manufacturing setting, understanding the individual performance of machines can lead to targeted interventions that improve productivity without disrupting the entire operation. Moreover, by analyzing employee productivity at a granular level, firms can identify training needs and enhance workforce performance.
- Resource Allocation: Businesses can allocate resources more effectively based on precise insights into operational needs.
- Cost Reduction: Identifying areas of waste or inefficiency helps companies to cut costs without compromising quality.
- Continuous Improvement: Microanalytics fosters a culture of continuous improvement. Organizations can constantly refine strategies based on real-time data.
Techniques in Microanalytics
In the realm of microanalytics, employing diverse techniques proves crucial for deriving meaningful insights from data. This section explores the various methods that can be leveraged to facilitate effective data analysis and informed decision-making. Understanding these techniques is essential because they help organizations extract value from data in a structured way. By employing both qualitative and quantitative approaches, businesses can gain a comprehensive view of their operational landscape. Each technique has its unique advantages that cater to different business needs.
Qualitative vs. Quantitative Approaches
When delving into microanalytics, differentiating between qualitative and quantitative approaches is vital. Qualitative approaches focus on understanding underlying reasons and motivations. They capture subjective experiences through methods like interviews, focus groups, and open-ended surveys. This data is usually descriptive, shedding light on patterns regarding customer behavior. For instance, customer feedback on a product may reveal insights about satisfaction levels or areas needing improvement.
In contrast, quantitative approaches emphasize numerical data and statistical analysis. This method benefits from structured data, often obtained through surveys with closed questions, web analytics, or transaction logs. With quantitative data, businesses can track performance metrics like sales conversion rates and user engagement.
Choosing between the two approaches often depends on the specific research question at hand. For example, when seeking to understand user preferences deeply, qualitative methods can provide richer context. Conversely, quantitative data is more effective for analyzing trends across large datasets and driving efficient decision-making.
Real-Time Data Processing
Real-time data processing is a key component of microanalytics, enabling businesses to obtain insights instantly. In today's fast-paced environment, the ability to analyze data as it is generated can greatly enhance responsiveness. Businesses equipped with real-time analytics can make informed decisions quickly, based on the latest information available.
Technologies like Apache Kafka and StreamSets allow for the seamless flow of data from various sources. This kind of data processing framework not only aids in immediate decision-making but also improves operational efficiency. For example, e-commerce platforms can adjust pricing dynamically based on customer behavior observed in real time.
However, implementing real-time processing requires careful consideration of IT infrastructure to ensure it can handle the continuous data stream effectively. Organizations must also invest in training for staff to utilize these advanced tools, ensuring they can interpret data accurately and act on it.
Data Segmentation Strategies
Data segmentation is essential for extracting actionable insights from vast datasets. This technique involves dividing data into distinct groups based on specific criteria. Effective segmentation allows businesses to target their marketing efforts, customize products, and improve customer satisfaction. For example, a retail store might segment customers based on their purchasing behavior.
Key strategies in data segmentation include:
- Demographic Segmentation: Categorizing data by characteristics such as age, gender, or income level.
- Behavioral Segmentation: Focusing on consumer behavior such as purchasing patterns and preparation times.
By utilizing data segmentation, businesses can achieve greater precision in their strategies. Targeted campaigns often yield higher engagement and conversion rates, as messages resonate more with specific audience segments. Yet, it is necessary for organizations to ensure they have the right tools and methodologies for ongoing analysis to adapt to evolving data patterns.
"Effective microanalytics techniques transform raw data into actionable insights, underpinning enhanced decision-making and operational efficiency."
Integration with Business Systems
The ability to integrate microanalytics with existing business systems is crucial for a seamless flow of information. This integration ensures that data from various departments, such as sales, marketing, and operations, can be analyzed together. As a result, it generates insights that are more comprehensive and actionable. Without integration, the value of microanalytics significantly diminishes. An organization may possess vast amounts of data, but if this data is not connected, it cannot be fully leveraged.
Some specific elements that underline the importance of this integration include:
- Real-time Data Access: Real-time access to data allows businesses to make swift decisions based on the most current information, significantly improving agility.
- Holistic View of Operations: Integrating systems provides a complete picture of business operations, leading to better strategic planning.
- Streamlined Processes: Automation most often accompanies integration, which can lead to reduced human error and increased efficiency.
Considerations during integration may include compatibility of existing software, resource allocation for implementation, and training employees on new systems. These factors can present hurdles that businesses need to plan around thoughtfully.
Linking Microanalytics to Existing Software
Integrating microanalytics with existing software can be seen as a bridge between raw data and meaningful insights. Existing software like customer relationship management (CRM) platforms and enterprise resource planning (ERP) systems often house a wealth of untapped data. By linking microanalytics directly to these platforms, organizations can unlock valuable insights without overhauling their current systems.
An effective connection can:
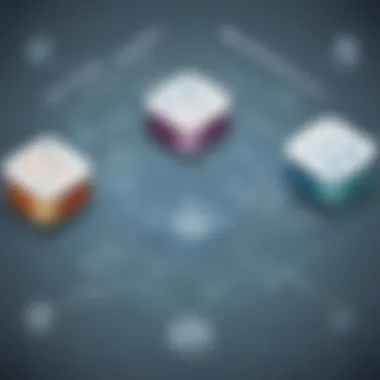
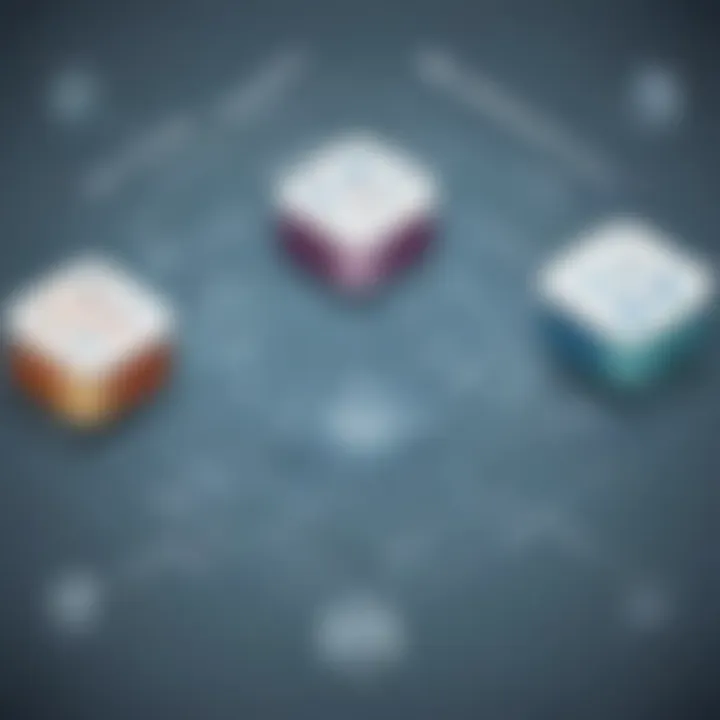
- Enhance Customer Insights: By linking microanalytics to CRM software, businesses can gain a deeper understanding of customer behavior and preferences.
- Monitor Key Performance Indicators: By integrating KPIs into analytics tools, organizations can have a better grasp on their performance.
Consider popular software solutions, such as Salesforce or HubSpot; these platforms can benefit significantly from microanalysis, providing data-driven insights that can enhance customer engagement strategies and operational decisions.
Creating a Unified Data Ecosystem
The creation of a unified data ecosystem is an essential outcome of successful integration. This ecosystem consists of interconnected systems that allow data to move freely and be accessed by all relevant stakeholders. It creates a landscape where data silos no longer exist, enabling data to be harnessed effectively for various purposes.
A unified data ecosystem can:
- Facilitate Collaboration: With easier access to shared data, teams across departments can collaborate more effectively, contributing to a more cohesive strategic vision.
- Drive Innovative Solutions: An interconnected ecosystem allows for the identification of patterns and trends that might not be visible when data is isolated.
“Efficiency is doing things right; effectiveness is doing the right things.”
To create such an ecosystem, organizations need to prioritize data governance, invest in compatible tools, and ensure rigorous training protocols. This high level of integration will support informed decision-making, essential for small to medium-sized businesses and entrepreneurs striving for growth and efficiency in a competitive market.
Impact on Business Performance
In the context of microanalytics, understanding its influence on business performance is critical for organizations seeking to enhance their decision-making processes. Microanalytics allows businesses to dissect data at a granular level, offering insights that are often overlooked by traditional analytics. This capability can lead to improved operational efficiency, more strategic resource allocation, and ultimately, a better bottom line.
Implementing microanalytics enables small to medium-sized enterprises to pinpoint areas for improvement with remarkable precision. For instance, businesses can analyze customer behaviors in real-time, allowing them to adapt their marketing strategies instantly. Moreover, this approach helps in identifying inefficiencies within operational processes, providing a clear path for optimization.
Some specific elements that contribute to the impact of microanalytics on performance include the following:
- In-Depth Customer Insights: Microanalytics provides detailed insights into customer preferences and behaviors, facilitating targeted marketing efforts.
- Real-Time Feedback: Organizations receive immediate feedback from their analytics, which enables them to make swift adjustments to their strategies.
- Data-Driven Culture: Adopting microanalytics fosters a culture where decisions are made based on empirical evidence as opposed to intuition.
In summary, the integration of microanalytics significantly enhances business performance by transforming how organizations make decisions based on data.
Case Studies of Successful Implementation
To illustrate the effectiveness of microanalytics, let’s examine a few case studies.
- Retail Sector - Target: Target corporation utilized microanalytics to analyze shopping patterns and personalize offers for its customers. By closely monitoring data, they improved customer engagement, which resulted in increased sales.
- Healthcare - Mount Sinai Health System: By implementing microanalytics for patient data, Mount Sinai improved treatment outcomes through personalized care plans. Their ability to analyze patient details led to enhanced operational efficiencies and reduced costs.
- Financial Services - American Express: American Express used microanalytics to better understand transaction patterns, allowing them to detect fraud more effectively. This significantly increased customer trust and reduced financial losses due to fraud.
These case studies illustrate not only the versatility of microanalytics across sectors but also underscore its capacity to drive significant performance improvements.
Analyzing Performance Metrics
Analyzing performance metrics is an integral aspect of microanalytics. Organizations must develop key performance indicators (KPIs) that align with their goals. By examining these metrics, businesses can quantify the effectiveness of their strategies and identify areas needing attention.
Focus areas include the following:
- Customer Satisfaction Scores: Understanding how satisfied customers are provides insights into product effectiveness and can highlight necessary changes.
- Conversion Rates: Monitoring conversion rates reveals how well marketing strategies resonate with target audiences and where adjustments may be necessary.
- Operational Efficiency Metrics: Metrics such as average handling time in customer service can indicate how effectively a team is operating.
Challenges in Adopting Microanalytics
As organizations increasingly recognize the potential of microanalytics, they face several critical challenges that can hinder effective implementation. Understanding these challenges is essential, as it helps businesses to navigate obstacles and leverage microanalytics for more informed decision-making. Key challenges include concerns over data privacy and technical barriers to better integration of systems. Addressing these issues not only safeguards organizational interests but also fosters a culture of data trust and operational excellence.
Data Privacy Concerns
In the current landscape, data privacy has taken center stage, impacting how companies gather, process, and analyze information. With regulations such as the General Data Protection Regulation (GDPR) in Europe and the California Consumer Privacy Act (CCPA), businesses must navigate complex legal frameworks. These laws impose stringent requirements on data collection and individual rights regarding their personal information.
Organizations that adopt microanalytics must ensure compliance with these regulations. This includes being transparent about what data is collected, how it is used, and who has access to it. Failure to comply can result in severe penalties and damage to the company’s reputation. Furthermore, engaging customers on this topic is paramount; stakeholders expect clear communication around data handling practices.
To address privacy concerns effectively, companies can take proactive steps:
- Implementing robust data governance policies.
- Using privacy-preserving techniques, such as encryption and anonymization.
- Conducting regular audits of data handling practices.
These measures not only protect individual privacy but also enhance customer trust, a vital component in today’s data-driven landscape.
Technical Barriers to Integration
Another significant hurdle for microanalytics adoption involves technical barriers related to integration with existing systems. Many organizations rely on various software solutions to manage their operations, and integrating microanalytics tools with these systems can be complex. This integration is often required for effective data utilization, yet can lead to compatibility issues and increased costs.
Moreover, the lack of standardized data formats across platforms often complicates the aggregation of insights. Employees might encounter difficulties accessing data needed for analysis. To overcome these barriers, companies should consider:
- Investing in training employees to improve technical skills that enhance integration efforts.
- Adopting solutions that offer seamless compatibility with existing systems.
- Engaging in change management strategies to ease transitions towards more integrated analytics workflows.
As organizations continue to adapt to the demands of a data-centric world, it becomes critical to carefully navigate these challenges. Recognizing the importance of data privacy and technical integration will empower organizations to fully harness the benefits of microanalytics, leading to improved operational efficiency and decision-making capabilities.
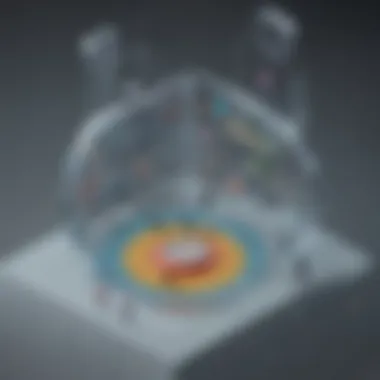
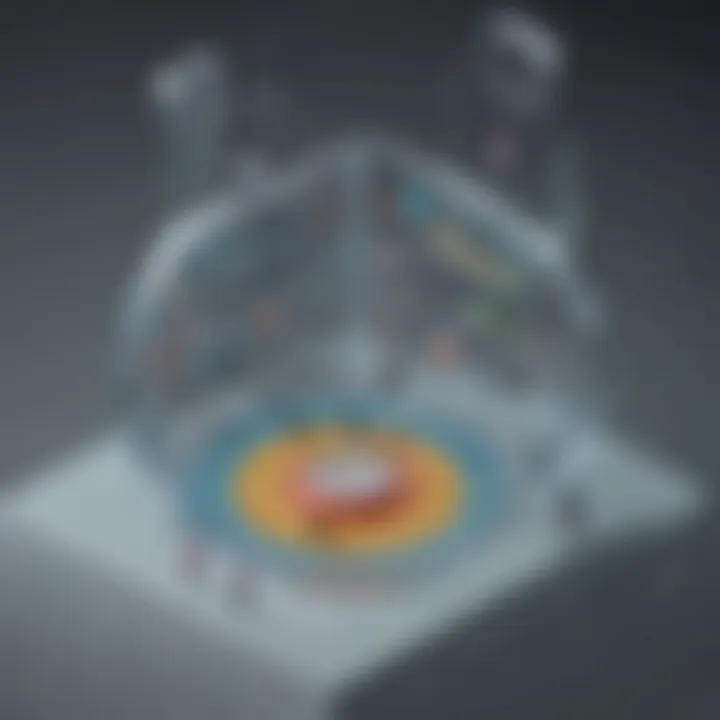
"Navigating the hurdles of microanalytics starts with understanding the landscape and implementing strategies that bolster data integrity and system compatibility."
By addressing these challenges head on, small to medium-sized businesses can position themselves favorably to capitalize on the insights offered by microanalytics, ultimately driving better business outcomes.
Ethical Considerations
Ethical considerations in microanalytics are paramount as businesses increasingly rely on data-driven decision-making. The responsible use of data can significantly influence trust between organizations and their stakeholders, including customers, employees, and partners. Poor data practices can lead to societal backlash and damage a company's reputation. Therefore, understanding how to navigate ethical dilemmas is essential for small to medium-sized businesses aiming to leverage microanalytics effectively.
Transparency in Data Use
Transparency in data use refers to open communication with stakeholders regarding how their data is collected, processed, and utilized. It fosters trust and promotes a cooperative environment. When businesses clearly articulate their data practices, they are more likely to gain stakeholder confidence, which can enhance customer loyalty.
Key elements of transparency include:
- Clear Consent: Informing users about what data is being collected and obtaining explicit permission.
- Accessible Policy: Providing easily understandable privacy policies that outline data usage.
- Regular Updates: Keeping stakeholders informed about any changes in data management practices.
By adopting transparent data practices, businesses can mitigate potential misunderstandings or conflicts over data usage. This not only safeguards customer relationships but can also ensure compliance with data regulations.
"Companies that prioritize transparency in their data practices often outperform competitors who do not, as trust translates into loyalty."
Responsible Data Management Practices
Responsible data management practices are essential in ensuring that data is handled with care and respect. This involves implementing measures that prioritize data security and privacy while maximizing the value gained from microanalytics. Key components include:
- Data Minimization: Collecting only essential data helps reduce exposure risks.
- Secure Storage: Employing advanced security measures to protect stored data from breaches.
- Regular Audits: Conducting periodic audits to ensure compliance with ethical standards and legal requirements.
Prompt action to remedy any identified weaknesses in data management can help organizations build robust frameworks that respect privacy while deriving insight from data analysis. Businesses must also provide training for employees on ethical data handling to align company practices with ethical standards.
In summary, the ethical considerations in microanalytics must not be an afterthought. Both transparency in data use and responsible management are crucial in enhancing operational efficiency and ensuring the trustworthiness of data-driven practices. With this commitment to ethics, organizations can foster an environment conducive to informed decision-making.
Future Trends in Microanalytics
Understanding the future trends in microanalytics is essential for businesses aiming to leverage data effectively. As technology evolves, microanalytics will continue to transform how decisions are made. This section will explore two primary trends: advancements in AI and machine learning and the rise of predictive analytics. Each has far-reaching implications for how organizations can harness data.
Advancements in AI and Machine Learning
The integration of artificial intelligence and machine learning into microanalytics is becoming pivotal. These technologies enable analysts to sift through vast datasets efficiently, revealing insights that were once hidden. AI can automate routine data collection and preprocessing tasks, allowing teams to focus on interpreting results rather than merely gathering data. This shift enhances operational efficiency and reduces the likelihood of human error in data handling.
Moreover, machine learning models can adapt over time. They learn from historical data to make real-time predictions. For small to medium-sized businesses, this means they can be more agile in their decision-making. They can respond swiftly to market changes based on predictive insights derived from data analysis.
However, businesses must remain conscious of the challenges that accompany these technologies. Implementing AI and machine learning requires a skilled workforce familiar with advanced technology. Organizations need to invest in training and development to equip their teams with the necessary skills to use these tools effectively.
The Rise of Predictive Analytics
Predictive analytics is another critical trend shaping microanalytics. This approach involves analyzing current and historical data to forecast future outcomes. By identifying patterns and trends, businesses can make informed decisions that anticipate changes in the market or consumer behavior. For instance, retail companies can utilize predictive analytics to optimize inventory management, ensuring that they restock popular items before they run out.
Additionally, predictive models can help businesses identify potential risks and opportunities. They can guide proactive interventions rather than reactive responses. This predictive capability enables organizations to stay ahead of the competition, aligning their strategies with anticipated market shifts.
"The future belongs to those who believe in the beauty of their dreams." - Eleanor Roosevelt
By focusing on these evolving trends, businesses can better position themselves to harness the power of microanalytics, driving more informed and effective decision-making.
Culmination and Implications for Businesses
Microanalytics has emerged as a pivotal component for small and medium-sized businesses seeking to refine their decision-making processes. This article emphasizes how microanalytics serves as a bridge between raw data and actionable insights. As organizations navigate an increasingly competitive landscape, the benefits of employing microanalytics cannot be overstated.
By implementing microanalytics, businesses harness the ability to make data-driven choices that are both timely and relevant. It is essential to acknowledge that these insights allow for improved targeting of marketing efforts, optimized resource allocation, and enhanced customer experiences. As a result, companies can adapt more swiftly to market trends and consumer behaviors.
Furthermore, the integration of microanalytics within existing operational frameworks ensures a smoother transition toward a data-centric culture. Small and medium-sized enterprises can leverage these insights without extensive resource investments.
This commitment to embracing microanalytics can set a business apart, driving innovation while ensuring sustainability in today's dynamic market.
However, it is crucial for organizations to remain aware of the challenges that accompany microanalytics adoption. Companies must prioritize data privacy and ethical considerations to maintain consumer trust. Failure to do so may lead to significant backlash that can tarnish an organization’s reputation.
Summarizing Key Takeaways
- Data-Driven Decision-Making: Microanalytics empowers businesses to base their choices on detailed insights rather than mere intuition.
- Cost-Effective Solutions: Implementing microanalytics can be done with minimal investment, making it accessible to smaller players in the market.
- Need for Ethical Approaches: With greater data usage comes the responsibility of managing it ethically, ensuring transparency and security in data handling.
- Integration is Key: Aligning microanalytics with current systems facilitates seamless adoption and maximizes the utility of collected data.
Future Directions for Research and Practice
As we look ahead, research in microanalytics is likely to evolve significantly. The rapid advancement of technologies like artificial intelligence and machine learning will expand the capabilities of microanalytics. This presents exciting opportunities for more robust data insights and predictive analytics.
Additionally, further study is needed on the impact of microanalytics across different industries. Examining successful implementations will guide new entrants and provide a framework for understanding best practices.
Moreover, organizations should focus on developing skills within their teams. Empowering employees to interpret data can lead to more informed decision-making. Training programs tailored to microanalytics’ competencies will be vital for sustaining business growth in an increasingly data-driven economy.