MonkeyLearn Sentiment Analysis: A Detailed Guide
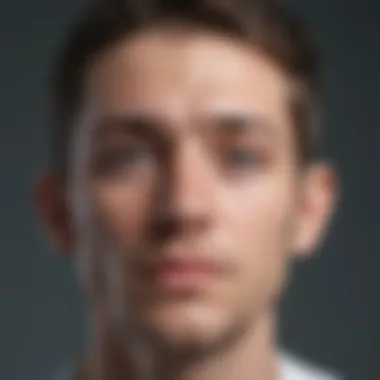
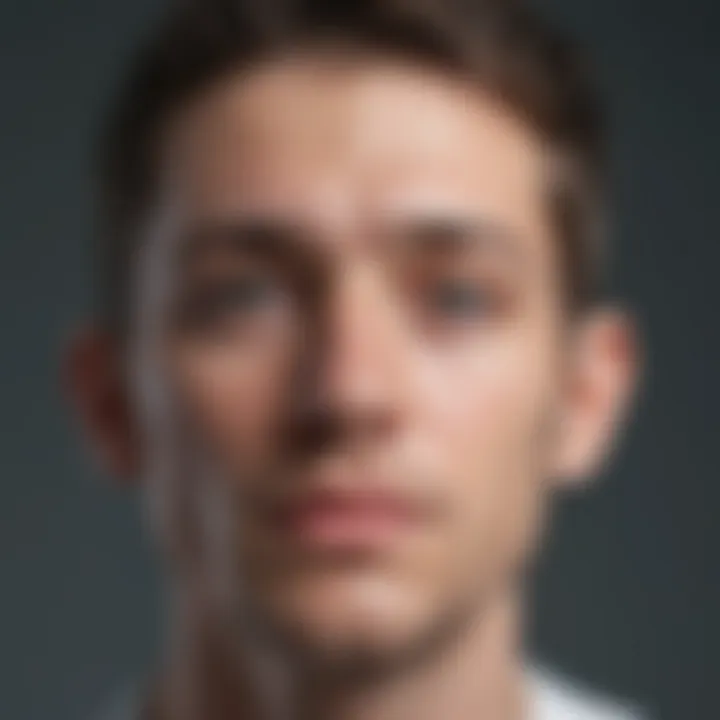
Intro
In today's ever-evolving digital landscape, businesses increasingly rely on data-driven insights to inform their strategies. MonkeyLearn, a prominent player in the field, has carved out a niche with its robust sentiment analysis capabilities. As companies bombard consumers with messages through various channels—be it social media, emails, or reviews—the need to decode feedback and gauge audience sentiment has never been more pressing. After all, understanding how your customers feel can be the difference between failure and success.
MonkeyLearn offers an accessible platform for small to medium-sized businesses and entrepreneurs looking to harness the power of sentiment analysis to improve decision-making. As we journey through this article, we will delve deeply into the various functionalities of MonkeyLearn, examine how these features might benefit users, and explore the software's unique selling points that potentially set it apart from the competition.
With that in mind, let’s begin by dissecting the cogs and gears that underpin MonkeyLearn’s feature set.
Overview of Features
Significant Functionalities
MonkeyLearn serves up a mix of features that make sentiment analysis not just another buzzword but a practical tool for organizational success. Here are some of the noteworthy functionalities:
- Text Classification: Users can categorize text data—be it from surveys, reviews, or social media posts—into predefined classes. This is particularly helpful in pinpointing customer feedback trends.
- Sentiment Analysis: The core of the software, this feature automatically determines whether a piece of text conveys positive, negative, or neutral sentiments. This feature can gauge customer satisfaction with pinpoint accuracy.
- Custom Models: Users have the flexibility to train their own models tailored to their specific needs, ensuring that the analysis is as relevant as possible to their unique context.
- API Access: For those equipped with tech-savvy teams, MonkeyLearn offers API functionalities for seamless integration into existing systems and workflows. This is an invaluable asset for continuous data analysis without interruptions.
Each of these features not only enhances operational efficiency but also empowers users to make informed decisions rooted in actual data.
Benefits to Users
The benefits of these functionalities extend beyond just surface-level insights. Here are a few ways users can capitalize on them:
- Enhanced Customer Insights: Gleaning actionable insights from user feedback can usher in strategic improvements in product offerings and customer service.
- Time-Saving Automation: By reducing the need for manual analysis, organizations can reallocate resources to other priorities, effectively increasing productivity.
- Data-Driven Decisions: With precise sentiment readings, businesses can pivot strategies quickly, keeping them ahead of competitors and in tune with market demands.
"Harnessing the power of sentiment analysis allows businesses to navigate customer feedback with clarity, driving smarter decisions and fostering growth."
Unique Selling Points
What Sets This Software Apart
In a crowded marketplace, it's hard to distinguish one sentiment analysis tool from another. However, MonkeyLearn has certain characteristics that elevate its stature:
- User-Friendly Interface: The platform is intuitive, meaning that even those with limited technical expertise can navigate it without breaking a sweat. This lowers the barrier for entry, letting businesses dive right in.
- Customization Options: Unlike many competitors that offer only cookie-cutter solutions, MonkeyLearn allows deeper customization, tailoring the experience to fit individual business needs.
- Integration Versatility: With a variety of integration options—ranging from direct API usage to compatibility with third-party tools—MonkeyLearn allows users to seamlessly incorporate sentiment analysis into their existing ecosystems.
Innovation and Specific Capabilities
MonkeyLearn illustrates a commitment to ongoing innovation. The platform continuously adapts to meet emerging trends and user needs. For instance, the incorporation of machine learning elements ensures that the analysis becomes more accurate over time.
By understanding and leveraging these unique selling points, businesses can truly maximize the potential of MonkeyLearn and foster an environment that values data-driven decision-making.
Understanding Sentiment Analysis
Sentiment analysis plays a pivotal role in deciphering the emotional tone behind a body of text, which can be crucial for businesses. With the vast amount of data generated daily, companies must harness these insights to stay ahead in a competitive landscape. This section delves into the essence of sentiment analysis, offering a clear lens through which readers can grasp its significance in today’s data-driven world.
Definition of Sentiment Analysis
Simply put, sentiment analysis is a sophisticated technique used to ascertain the sentiment expressed within a piece of text. This analysis can be broken down into three main categories: positive, negative, and neutral. By applying algorithms to analyze words and phrases, sentiment analysis sheds light on the underlying emotions conveyed through language. For instance, a social media post might express joy when a customer delivers a glowing review about a product, while a vague complaint could signal disappointment. Understanding these nuances is vital for tailoring responses and strategies.
Importance in Business
In the realm of business, knowledge is power and sentiment analysis is a formidable tool for gathering actionable insights. Companies that effectively leverage sentiment analysis can:
- Enhance Customer Engagement: Understanding how customers feel about products or services enables businesses to engage them on a more personal level.
- Refine Marketing Strategies: By gauging public sentiment, marketing teams can better tailor their campaigns to resonate with their audience.
- Monitor Brand Health: Tracking sentiment over time gives firms a pulse on their brand reputation, allowing them to address issues proactively.
This is more than just about numbers; it’s about human connection. Fostering a deeper understanding of customer perceptions can yield a unique advantage in an ever-evolving market.
How Sentiment Analysis Works
The mechanics of sentiment analysis can seem complex, but at its core, it involves several key components. Algorithms categorize text based on linguistic patterns and training data. Here’s how it typically unfolds:
- Data Collection: Text data is gathered from various sources such as social media, reviews, and blogs. This pool of information serves as the foundation for analysis.
- Text Processing: Algorithms filter the text to remove any noise, focusing only on words and phrases that contribute to sentiment.
- Model Training: The machine learning model is trained using labeled data, learning to predict sentiment based on existing patterns.
- Prediction: Once trained, the model can analyze new text inputs, categorizing them into positive, negative, or neutral sentiment.
The power of sentiment analysis lies in its ability to sift through vast amounts of text swiftly, generating insights that would take humans a significant amount of time to uncover manually. This efficiency is precisely what businesses need to respond to customer sentiments in real-time and adapt to market dynamics.
Prolusion to MonkeyLearn
Understanding what MonkeyLearn is can be pivotal for those looking to harness the power of sentiment analysis in a business context. The platform stands out as a user-friendly tool tailored for companies wanting to dive into the depths of text data. Connecting customer feedback to effective decision-making is no small feat, and that’s where MonkeyLearn comes into play.
Overview of the Platform
MonkeyLearn is an intuitive and robust platform designed to facilitate text analysis through its various tools catering to sentiment analysis. It enables businesses to extract insights from textual data effortlessly. Users can import data from sources such as social media, surveys, and reviews, allowing for a holistic view of customer sentiment towards their brands.
With a visual dashboard at its helm, navigating through data becomes second nature. Whether you’re an IT professional needing precise analytics or a small business owner just trying to understand your clientele better, MonkeyLearn makes it accessible.
Core Features of MonkeyLearn
The features of MonkeyLearn are crafted to meet diverse business needs:
- Sentiment Analysis: At its core, this assesses whether feedback is positive, negative, or neutral, offering a glimpse into customer emotions.
- Custom Models: Users can create tailored models, making it adaptable to specific industry lingoes or unique product feedback.
- Data Integrations: Smooth integration with various platforms like Zapier allows users to pull data from multiple sources seamlessly.
These features not only equip businesses with analytical tools but also ensure they remain agile in responding to market trends.
Target User Base
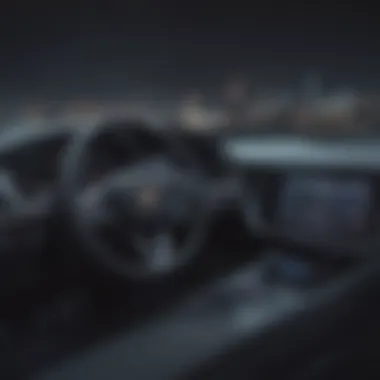
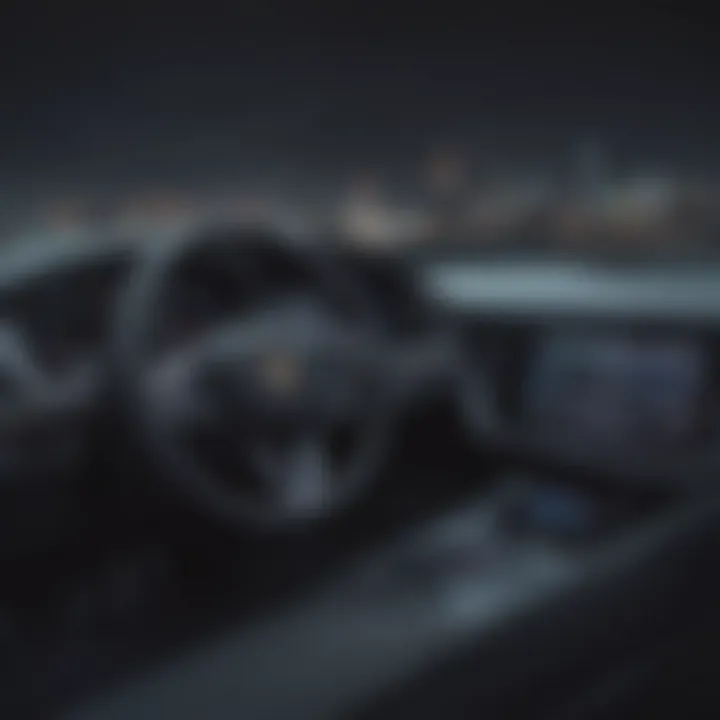
MonkeyLearn caters to a broad spectrum of users, focusing primarily on small to medium-sized businesses, entrepreneurs, and IT professionals. Here’s why these groups find value in the platform:
- Small to Medium-Sized Businesses: They often lack the resources for comprehensive analytics teams. MonkeyLearn safely bridges that gap, putting powerful sentiment analysis in their hands.
- Entrepreneurs: Understanding market sentiment can shape product development and marketing strategies.
- IT Professionals: They look for detailed, nuanced insights from text data to drive technical decisions and improve product offerings.
In a nutshell, MonkeyLearn equips its targeted users with the tools and insights necessary to enhance their business strategies.
The Mechanics of MonkeyLearn Sentiment Analysis
Understanding the mechanics behind MonkeyLearn's sentiment analysis tools is crucial for leveraging its full potential. This segment dives into the framework of natural language processing techniques, model training methodologies, and mechanisms for addressing sentiment ambiguities. For small to medium-sized businesses and IT professionals, these elements act as cornerstones for interpreting data accurately, thus enabling informed decision-making.
Natural Language Processing Techniques
Natural Language Processing (NLP) serves as the backbone of sentiment analysis. By dissecting human language through computational methods, MonkeyLearn helps parse through vast amounts of text efficiently. The platform utilizes various NLP techniques, including:
- Tokenization: Breaking down sentences into individual words or phrases to grasp the context better.
- Part-of-Speech Tagging: Identifying verbs, nouns, and adjectives to understand sentiment-bearing words.
- Sentiment Scoring: Assigning numerical values to detected sentiments, helping gauge positive, negative, or neutral responses.
These processes allow MonkeyLearn to analyze customer feedback, social media posts, and more, transforming raw data into useful insights.
Using NLP not only amplifies accuracy but also enhances the speed of analysis. This speed is vital for businesses looking to respond rapidly to shifts in public sentiment or emerging trends.
Model Training and Prediction
Model training is pivotal for ensuring that sentiment analysis generates reliable predictions. MonkeyLearn employs various machine learning algorithms to train its models on labeled data sets, effectively learning from examples.
The core steps in model training include:
- Data Collection: Gathering substantial volumes of labeled data that showcase different sentiment types.
- Feature Extraction: Identifying essential features from the data to assist the model in making accurate predictions.
- Training Phase: Delivering collected data to the model, allowing it to learn and adjust parameters.
- Testing and Validation: Assessing the model with unseen data to ensure it performs accurately across varied contexts.
This robust training process means that once the model is operational, it can make real-time predictions with commendable accuracy. This capability directly benefits businesses, providing them with timely insights that align with strategic objectives.
Handling Ambiguity in Sentiment
One of the complexities in sentiment analysis is dealing with ambiguous phrases. Human language is riddled with nuances, sarcasm, and context-dependent meanings, making straightforward interpretations challenging. MonkeyLearn tackles this issue head-on by incorporating advanced contextual analysis capabilities.
Factors that enhance handling ambiguity include:
- Contextual Training: Models that are trained to consider the context surrounding words, thus improving accuracy on ambiguous phrases.
- Sentiment Thesaurus: Including synonyms and contextual variations of words to broaden sentiment evaluation.
- User Feedback Loop: Allowing users to provide feedback on sentiment interpretations, continuously refining the models' accuracy.
By addressing ambiguity, MonkeyLearn's sentiment analysis offers businesses a clearer understanding of the data at hand, making it easier to capture sentiments accurately.
"The nuances in human language make sentiment analysis both an art and a science. MonkeyLearn bridges that gap effectively."
Overall, the mechanics of MonkeyLearn's sentiment analysis provide a robust framework for extracting insights from unstructured data. By integrating advanced NLP techniques and sophisticated model training methods, organizations can navigate the complex terrain of human emotions captured in text, ultimately driving better business decisions.
Applications of Sentiment Analysis in Business
Sentiment analysis stands out as an essential tool for businesses keen on harnessing the power of consumer feedback. As companies face an ever-evolving landscape of customer expectations and feedback platforms, understanding sentiment becomes not just a benefit but a necessity. By translating emotions captured in text data into actionable insights, businesses can steer their strategies with greater precision. This section explores how various elements of sentiment analysis can reshape business practices, enhance consumer engagement, and ultimately influence bottom lines.
Market Research and Consumer Insights
In the fast-paced world of consumer goods, businesses thrive on timely and accurate insights. Sentiment analysis serves as a goldmine when it comes to market research. Companies can sift through consumer comments, social media interactions, and survey responses to grasp public opinion on products or services. This analysis helps understand not only what consumers say but also how they feel.
- Consumer Trends: By monitoring changes in sentiment over time, businesses can identify emerging trends and adapt their offerings accordingly. This enables them to stay ahead of the game.
- Targeted Marketing: Insights drawn from sentiment analysis allow organizations to tailor their marketing messages to resonate with specific audience segments. If the feedback indicates that consumers are leaning towards sustainability, companies can showcase their eco-friendly practices in marketing campaigns.
Utilizing sentiment analysis tools, businesses can establish a clear picture of how their audience perceives them, leading to more informed decisions.
Product Development Feedback
When it comes to product development, understanding sentiment is crucial. Feedback is often the bridge between a concept and a successful product launch. Analyzing sentiment allows companies to tap into the voice of the customer right at the product planning stage.
- Feature Enhancements: Companies can prioritize new features or improvements based on what customers are asking for or complaining about, ensuring that the end product aligns closely with market demands.
- Prototype Testing: Early sentiment analysis helps gauge reactions to prototypes, offering critical insights into potential consumer reception. This preemptive approach can save time and resources down the line.
In essence, sentiment analysis acts as an ongoing dialogue with consumers, guiding the development process to ensure products hit the mark every time.
Brand Reputation Management
In today’s digital age, a brand’s reputation can change overnight, often driven by consumer sentiment expressed through social media or review sites. Monitoring sentiment is vital for companies looking to maintain their reputation and respond swiftly to potential crises.
- Real-Time Alerts: Some sentiment analysis tools provide real-time alerts about negative spikes in sentiment. This allows businesses to intervene before situations escalate.
- Community Engagement: Understanding sentiment helps brands know how to engage with their audiences. If feedback is leaning negative, a well-strategized response can rebuild trust and loyalty.
Thus, actively managing sentiment not only protects brands but can also enhance goodwill within their communities, turning potential crises into opportunities for growth.
Key takeaway: The integration of sentiment analysis into business practices isn’t just about collecting data—it’s about fostering relationships and making informed decisions.
Integrating MonkeyLearn with Existing Systems
Integrating MonkeyLearn with current systems is a crucial step for businesses aiming to leverage sentiment analysis effectively. The ability to blend this powerful tool with existing infrastructure allows organizations to enhance decision-making capabilities without overhauling their operational framework. When it comes to integrating MonkeyLearn, several factors deserve consideration, including cost-effectiveness, efficiency, and user experience.
API Functionality and Customization
MonkeyLearn provides a robust API that offers powerful customization options tailored for individual business needs. This allows organizations to automate workflows and streamline data processing with relative ease. For instance, a marketing team can set up a system where user feedback from social media channels is gathered, analyzed, and reported automatically via the API, leading to quicker adjustments in strategy. Here are a few key aspects of the API functionalities:
- Robust Data Handling: You can send bulk data for analysis, receiving structured sentiment results in return. This facilitates high-volume analysis crucial for businesses with large datasets, like e-commerce sites.
- Real-Time Processing: The API allows for real-time sentiment analysis, which can be vital for timely decisions, particularly during product launches or crisis management.
- Integration with Other Tools: Customizable API endpoints can easily connect with tools like Slack for internal communications, or Salesforce for customer relationship management, enhancing overall operational effectiveness.
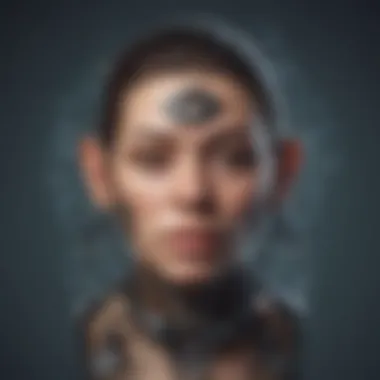
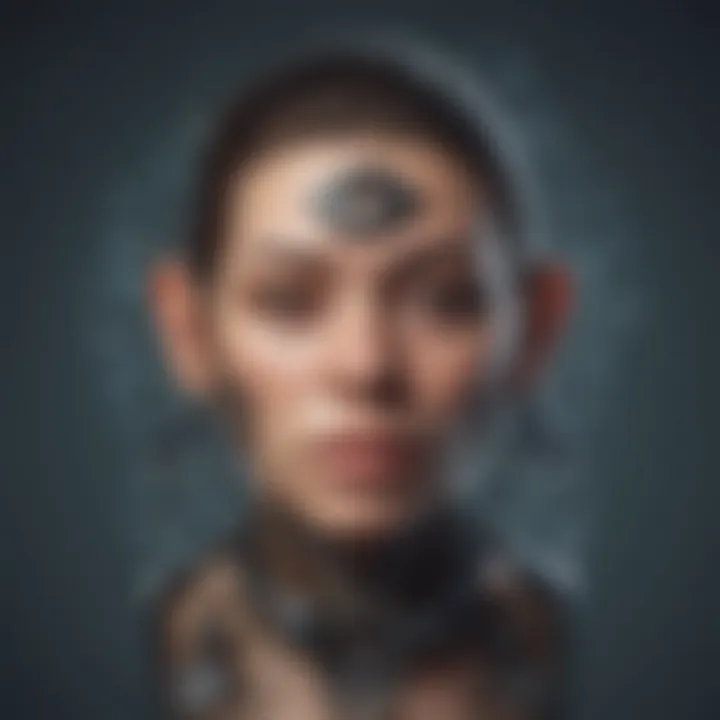
With these functionalities, the integration process becomes less of a headache and more of a streamlined experience, allowing for enhanced adaptability.
Compatibility with Other Software Solutions
When considering any new tool, having it play nice with existing programs is paramount. MonkeyLearn does not fall short here. Its design ensures compatibility with a plethora of other software solutions prevalent in the business landscape. Whether it’s CRM, email marketing platforms, or project management tools, businesses can find ample ways to mesh MonkeyLearn with what they already utilize.
- Gestalt of Integration: The seamless integration reduces the learning curve for employees, enabling them to use both MonkeyLearn and their existing systems in tandem without much fuss.
- Expansion of Analytics Capabilities: By linking with databases like MySQL or cloud services such as Google Cloud, you can expand your analytics capacity, enabling in-depth dives into data correlations.
- Ease of Accessibility: With existing tools, teams can access data from various platforms without switching contexts, thus improving productivity as they analyze sentiment data directly in environments they are already familiar with.
Best Practices for Integration
Integrating a tool like MonkeyLearn isn't just plug-and-play; a few best practices can help organizations maximize the return on their investment:
- Plan Strategically: Before diving into integration, map out the specific goals you intend to achieve with sentiment analysis. Defining these beforehand will guide the customization and deployment process.
- Training and Support: Consider providing training sessions for teams that will use MonkeyLearn to familiarize them with how it functions in conjunction with existing tools. A well-informed team will utilize the tool to its fullest potential.
- Monitor Performance: After integrating, keep a close watch on how the system is performing. Regular evaluations not only track sentiment accuracy but also identify areas for further improvement.
- User Feedback Loop: Create a channel for users to provide feedback on the integration process itself. Their insights can aid in refining the approach, making future integrations smoother.
"Integration is not just an action—it's a journey that shapes your sentiment insights into actionable advice for business growth."
By following these best practices, businesses can not only ensure a smoother integration process but also enhance the potential benefits gained from MonkeyLearn's sentiment analysis capabilities. Enhancing the decision-making process through thoughtful integration can result in informed, proactive strategy shifts that align with overall business objectives.
Comparative Analysis: MonkeyLearn vs. Other Tools
Understanding how MonkeyLearn stacks up against other sentiment analysis tools is crucial for organizations looking to harness the power of data-driven insights. A comparative analysis not only highlights the features and functionalities of MonkeyLearn but also illuminates where it stands in the competitive landscape. This section will delve into the competitors in the sentiment analysis arena and analyze MonkeyLearn's strengths and weaknesses.
Key Competitors in Sentiment Analysis
The sentiment analysis tool market is crowded with various options, each claiming to provide unique advantages. Here are some notable competitors:
- Lexalytics: This tool is known for its natural language processing capabilities and caters primarily to large enterprises. It provides comprehensive text analytics with a strong focus on customizable sentiment detection.
- Sentiment140: Aimed specifically at analyzing Twitter data, this tool is straightforward and provides insights based on public sentiment towards brands or events. Its niche focus makes it a popular choice for social media sentiment analysis.
- IBM Watson Natural Language Understanding: Part of IBM's AI suite, this solution provides sentiment analysis among a variety of NLP capabilities. It is robust but can be complex due to the numerous features offered.
- Hootsuite Insights: Built primarily for social media monitoring, Hootsuite Insights incorporates sentiment analysis into its platform. Its integration with social media channels is a significant draw for businesses focused on online reputation management.
- Google Cloud Natural Language API: This is a comprehensive program allowing businesses to analyze text for sentiment across vast datasets. It’s widely recognized but can require more technical expertise for effective use.
Identifying these tools allows companies to weigh their options carefully. By understanding the strengths of each competitor, firms can pinpoint which tool aligns with their specific needs and goals.
Strengths and Weaknesses of MonkeyLearn
MonkeyLearn brings a tailored approach to sentiment analysis, which is evident in its unique features and functionalities. However, like any tool, it has both advantages and some limitations.
Strengths:
- User-Friendly Interface: MonkeyLearn’s platform is designed with non-technical users in mind. This ease of use allows small to medium-sized businesses to dive into sentiment analysis without needing extensive training.
- Customization Options: The ability to customize sentiment analysis models allows businesses to tailor the tool to their specific industry or company language. This flexibility can enhance the accuracy of insights generated.
- Integration Capabilities: MonkeyLearn can easily integrate with other applications through its well-documented API, making it a convenient choice for businesses looking to embed sentiment analysis into existing workflows.
- Real-Time Analysis: The capability to analyze incoming data instantly is vital for businesses, especially in crisis management situations. MonkeyLearn enables timely insights that can influence immediate decision-making.
Weaknesses:
- Scalability Concerns: While MonkeyLearn is well-suited for small to medium-sized enterprises, larger organizations may find the tool needing more robust features for extensive data processing tasks.
- Limited Advanced Functions: Compared to high-end tools like IBM Watson, MonkeyLearn's advanced options for deep learning and analysis could be seen as lacking by companies requiring more complex functionalities.
- Cost Considerations: For some businesses, the subscription and pricing model may pose limitations, especially if users seek more extensive features found in competitor pricing schemes.
Conducting a comparative analysis helps businesses make informed decisions. By weighing the strengths and weaknesses of MonkeyLearn against its competitors, organizations can better understand which sentiment analysis tool may best serve their needs.
"Choosing the right sentiment analysis tool can make or break how you interpret customer emotions and feedback. Assessing your options objectively is key to success."
Case Studies: Successful Implementations
Exploring real-world examples of how sentiment analysis has been effectively integrated into business practices serves two crucial purposes. First, it illustrates the profound impact that sentiment insights can have on decision-making. Second, it supplies practical lessons for businesses looking to leverage MonkeyLearn's capabilities. By dissecting these case studies, we uncover not just success stories but also thoughtful strategies that can help organizations navigate the complexities of sentiment analysis.
Industry-Specific Applications
Sentiment analysis is a versatile tool, applicable across diverse industries. Here are some notable cases showcasing its adaptability:
- Retail Sector: A leading clothing retailer utilized MonkeyLearn to analyze customer reviews and feedback from social media platforms. By categorizing sentiments around certain products, they were able to optimize their inventory, tailoring their offerings to better align with customer desires. For instance, the retailer identified a trend indicating a preference for eco-friendly items, leading to an increase in sales of sustainable clothing lines.
- Healthcare: A hospital leveraged sentiment analysis to assess patient feedback from various digital channels. The insights gleaned highlighted areas needing improvement, such as patient wait times and service quality. Post-implementation of changes based on this data, the hospital reported a 25% increase in patient satisfaction scores.
- Finance: A financial services firm employed sentiment analysis to monitor brand reputation in real-time, particularly during financial market fluctuations. By understanding public sentiment, they adapted their communication strategy, which allowed them to mitigate negative public perception during rough patches in the market.
By utilizing MonkeyLearn, these organizations not only understood their clientele better but also drove their strategies with a data-backed approach, enhancing customer experience and operational efficiency.
Lessons Learned from Users
Engaging with users who have effectively applied sentiment analysis reveals valuable lessons. It is critical to recognize specific takeaways that can apply to any business considering this technology:
- Start Small, Scale Gradually: Many users recommend beginning with a clear focus on a limited scope, such as monitoring feedback on specific product lines. As proficiency increases, expansions into broader datasets can happen.
- Tailor Your Models: Users stressed the importance of customizing sentiment analysis models to cater to the unique language and context of their particular industry. Generic frameworks might not capture the nuances in customer feedback, leading to skewed insights.
- Regularly Update Models: Language evolves, and feedback trends shift. Businesses must continuously refine their sentiment analysis models to remain relevant. Regular retraining of models based on the latest data ensures the insights stay sharp and actionable.
- Engage with the Results: Simply collecting sentiment data isn’t enough. Engaging stakeholders across departments—marketing, product development, customer service—ensures that insights are effectively integrated into organizational strategies. This cross-departmental collaboration can unveil further opportunities to leverage sentiment analysis.
"Data is not just numbers; it tells a story. What we need to do is listen carefully to that story and act accordingly."
These insights illustrate that successful implementation of sentiment analysis isn’t solely about the technology itself. It requires a holistic approach that integrates data insights into the core decision-making processes of the business. Understanding these lessons not only helps other organizations avoid pitfalls but also paves the way for richer, more nuanced engagement with customers.
Challenges in Sentiment Analysis
Sentiment analysis holds a prominent spot in today's data-driven landscape. However, businesses looking to harness the power of sentiment tools like MonkeyLearn face multiple hurdles. A solid understanding of these challenges is crucial for small to medium-sized businesses, entrepreneurs, and IT professionals to maximize the effectiveness of their sentiment analysis efforts.
Data Quality and Availability
For sentiment analysis to work seamlessly, the foundation of any successful project is data quality. If the data going into the analysis is of poor quality or sparse, the results can be misleading. Sometimes companies rely on user-generated content, which could be rife with inconsistencies and noise. You might find even well-established brands are not immune to this issue; for instance, consider how product reviews can veer off-topic or be exaggerated.
- Why It Matters: Poor data quality can lead to incorrect sentiment assessments, affecting strategic decisions.
- Availability: Even if you have access to massive datasets, without an accurate representation of your audience's feelings or opinions, the results lose their value.
To combat these challenges, organizations should focus on curating data sources carefully, ensuring they reflect the authentic voice of their customers. Validated data sources enhance not only the accuracy of the insights but also the trust in automated outcomes.
Contextual Limitations
Navigating the nuances of language can often feel like walking through a dense fog. People express their opinions and sentiments differently based on various contexts. A statement that appears positive in one situation might have a negative undertone if the context shifts slightly. Consider a phrase like "This product works sometimes". Ideally, it sounds good, but in reality, the sentiment could very well be lukewarm at best.
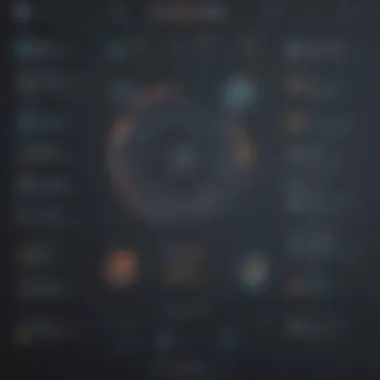
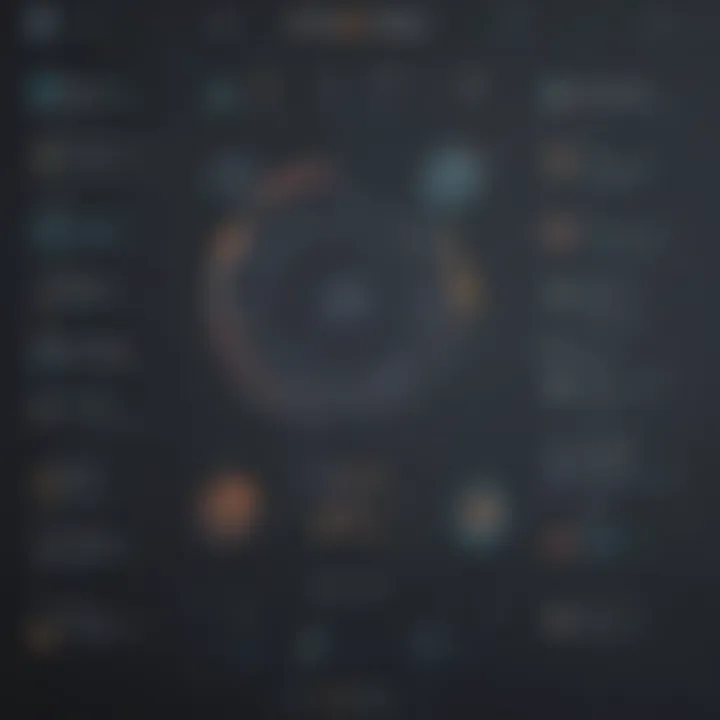
- The Challenge: Ambiguities arise not just from the words used but also from the overall context in which they’re delivered.
- Implications: Sentiment analysis tools might take things at face value, leading to under or overestimating customer sentiment.
To address contextual limitations, businesses should incorporate sophisticated natural language processing techniques. Using tools that allow for deeper insights into context can help mitigate superficial interpretations and result in more accurate sentiment profiles.
Evolving Language and Sentiment
Language is ever-evolving, and with this change comes the challenge of keeping up. New slang, idioms, and even emojis seem to pop up overnight. The language you see in social media is quite different from formal communication, and understanding the sentiment behind these variations requires adaptability. Notably, a simple emoji can change the entire sentiment conveyed in a message.
- Keeping Pace: Companies need to ensure that their sentiment analysis models are frequently updated to understand these changes.
- Cultural Context: What may be perceived as positive in one culture could be interpreted negatively in another, making it necessary to consider global audiences in sentiment modeling.
"A program that can't understand new slang is as useful as a car without fuel."
Unless your tools adapt to language evolution, insights become stale and potentially misleading. It's vital for organizations to maintain a proactive approach by regularly training models on current data, focusing on trends and changes in language usage.
Addressing these challenges directly not only helps in refining the sentiment analysis process but also empowers organizations to use data-driven strategies effectively. The crux lies in understanding that while sentiment analysis provides valuable insights, being aware of its limitations can truly harness its potential.
Future Trends in Sentiment Analysis
As we look ahead, the landscape of sentiment analysis is evolving at breakneck speed. The significance of understanding sentiment trends cannot be overstressed, especially for businesses seeking to harness customer insights for strategic advantage. With advancements in technology, these trends are set to transform how organizations engage with consumers and process feedback. While some businesses might still be wading through the basics, others are gearing up to ride the wave of innovation.
Advances in Machine Learning
Machine learning is at the core of sentiment analysis improvements. This technology fuels algorithms that learn from vast amounts of data, enhancing their predictive capabilities over time. Recent trends include:
- Deep Learning: Methods such as recurrent neural networks (RNNs) are playing a crucial role in understanding context better. They help analyze sequences of words and grasp nuances like sarcasm much more accurately.
- Transfer Learning: This technique allows one to leverage pre-trained models on similar tasks, saving time and resources while improving performance. For instance, models pre-trained on extensive datasets can be fine-tuned for specific domains like finance or health.
- Real-time Analytics: The ability to analyze sentiments in real time is becoming imperative. Trends indicate a shift toward integrating real-time analysis, facilitating immediate responses to customer emotions, which can dramatically enhance customer engagement.
Integration with Other AI Technologies
Sentiment analysis no longer exists in isolation. Today's tools often integrate with other artificial intelligence technologies, augmenting their effectiveness. Consider the following:
- Natural Language Processing (NLP): Combining sentiment analysis with advanced NLP techniques improves the understanding of subtleties in language, helping businesses capture more meaningful insights from customer feedback.
- Chatbots and Virtual Assistants: By embedding sentiment analysis in chatbots, companies can tailor responses based on a user's emotional state, thus creating a more personalized interaction.
- Predictive Analytics: Sentiment tools can integrate with predictive analytics to forecast future trends. This synergy allows businesses to strategize based on not just current sentiments but also anticipated market movements.
Impact on Business Strategy
The shifts in sentiment analysis technology are poised to substantially impact business strategies. Here’s how:
- Data-Driven Decision Making: Companies are increasingly using sentiment data to drive their decision-making processes. With a clear understanding of customer sentiment, organizations can streamline product development and marketing strategies more effectively.
- Enhanced Customer Experience: By acting swiftly on sentiment data, businesses can boost customer satisfaction. Addressing negative sentiments before they escalate can foster loyalty.
- Competitive Advantage: Businesses that adopt these trends early often gain a leg up on competitors. Utilizing advanced sentiment analysis helps in positioning products or services more strategically in the market, based on customer feedback.
"As sentiment analysis continues to evolve, the potential for businesses to respond to the customer landscape dynamically cannot be overlooked. Proactive engagement informed by sentiment can redefine customer relationships."
In summary, future trends in sentiment analysis are not just about refining existing technologies, but also about redefining how businesses perceive and react to their customer's feelings and opinions. As these advancements unfold, they hold the promise of enabling organizations to navigate customer sentiments more astutely, ensuring a robust and resonant connection with their audience.
Ethical Considerations in Sentiment Analysis
The landscape of sentiment analysis is intricate, shedding light on consumer emotions and perceptions. However, wrapped within its potential lies the necessity for a careful ethical approach. Navigating these waters is particularly important for small to medium-sized businesses, entrepreneurs, and IT professionals who are keen to leverage such insights without crossing ethical lines. Understanding the ethical landscape can bolster reputation, foster trust, and ultimately lead to better engagement with consumers.
Incorporating sentiment analysis into business operations opens up doors to improved decision-making, yet this must be balanced with vigilance regarding privacy and biases embedded within the analytics.
Data Privacy and Consent
Data privacy stands out as a pressing concern in today’s digital world. For sentiment analysis to function effectively, organizations require access to user-generated data, whether it's from social media platforms, customer feedback forms, or any other type of interaction. This data often carries personal information that can be sensitive in nature.
Organizations must ensure that they are compliant with legal frameworks such as GDPR or CCPA, which emphasize transparency and consent. This translates to:
- Obtaining explicit consent from users before utilizing their data.
- Clearly defining how the data will be used and processed.
- Offering users options to opt-out and manage their privacy settings.
By doing so, a business can not only adhere to legal standards but also reinforce trust with its clientele. Customers are likely to engage more when they’re confident that their data is handled responsibly and transparently.
Bias in Sentiment Models
Bias is an insidious issue in the realm of sentiment analysis, often lurking within the data models used to derive insights. If training datasets are not diverse enough, the outcomes can favor specific demographics or perspectives, distorting the actual sentiment reflected in the broader population.
This creates challenges such as:
- Misinterpretation of brand sentiment.
- Ineffective marketing strategies aimed at skewed audience segments.
- Alienation of group identities not represented in the data.
Thus, it’s vital for businesses to take a proactive stance in ensuring that their sentiment models are trained on diverse datasets. Regularly auditing these models and making adjustments can help mitigate biases. Companies should foster an environment where diverse voices can be heard and represented in their sentiment analysis efforts.
Ethical considerations aren't just about compliance; they represent the essence of responsible business practices that build customer loyalty and trust.
By addressing data privacy and bias, organizations can enhance their credibility and ensure that their use of sentiment analysis evolves harmoniously with societal values.
Closure
The conclusion wraps up the exploration of MonkeyLearn's capabilities in sentiment analysis, presenting a holistic view of its potential within the business landscape. It serves not just as a summary, but as a crucial capability for businesses to understand sentiments from data gathered through various channels. An effective sentiment analysis tool like MonkeyLearn can enhance strategic decision-making by providing meaningful insights that go beyond mere numbers.
Recap of Key Insights
In reviewing the content discussed throughout the article, several key points emerge:
- Importance of Sentiment Analysis: Sentiment analysis is vital for gauging consumer feelings and reactions towards products and services. Understanding customer sentiment can directly influence marketing strategies and product development.
- MonkeyLearn’s Features: The platform stands out due to its user-friendly interface, robust API functionalities, and customizable options that cater to a variety of business needs.
- Applications in Business: Real-world applications such as market research, customer feedback, and brand management illustrate how companies leverage sentiment analysis for a competitive edge.
- Integration Challenges: While the integration of sentiment analysis tools can be powerful, challenges such as data quality and contextual limitations must be addressed for effective implementation.
This recap emphasizes the importance of understanding not just what sentiment analysis is, but how it can be strategically applied within various business contexts.
Final Recommendations for Businesses
To maximize the benefits of sentiment analysis using MonkeyLearn, businesses should consider the following:
- Invest in Training: Proper training on how to utilize the platform's features is crucial for employees. This reduces the learning curve and enhances productivity.
- Monitor and Optimize: Regularly evaluate the outputs from sentiment analysis. If results are not aligning with business goals, adjust the parameters or retrain models.
- Focus on Data Quality: Ensure that the data fed into the system is clean and relevant. Quality data leads to more accurate insights, which ultimately supports better decision-making.
- Stay Updated on Trends: As the landscape of sentiment analysis evolves, staying informed about the latest developments in AI and machine learning technologies will keep your business ahead of the curve.
By applying these recommendations, small to medium-sized businesses, entrepreneurs, and IT professionals can leverage MonkeyLearn’s sentiment analysis more effectively and drive meaningful outcomes.