Understanding Databricks SKU for Business Success
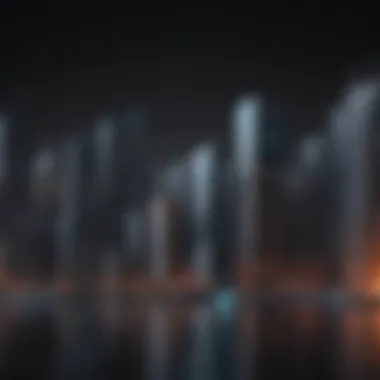
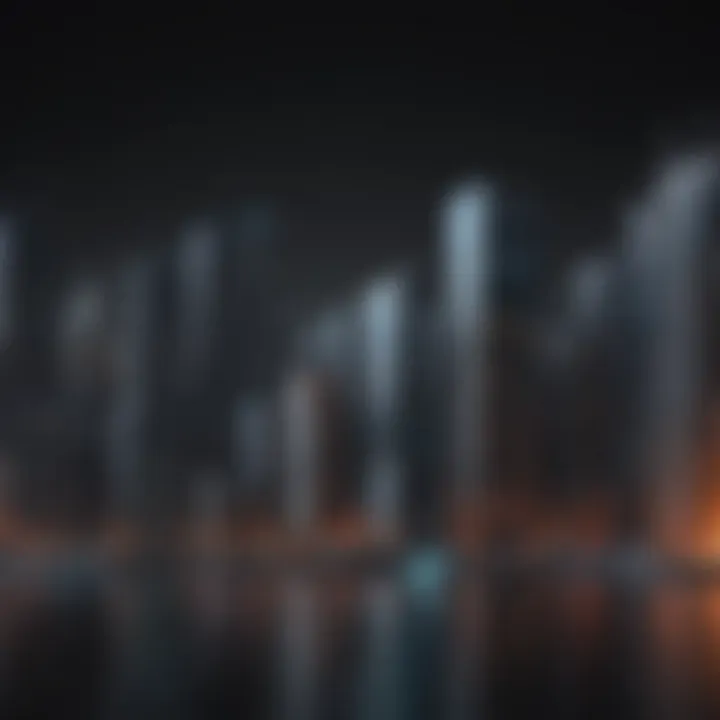
Intro
In the current landscape of digital transformation, businesses face numerous challenges related to data management and analysis. Databricks, a data and AI platform built on Apache Spark, is increasingly recognized for its capabilities in these areas. Understanding the SKU or Stock Keeping Unit structures of Databricks is crucial for enterprises looking to optimize their data strategies. This guide aims to provide clarity on the features, advantages, and various deployment models of Databricks SKU, focusing specifically on how it benefits small to medium-sized businesses and IT professionals.
Overview of Features
Databricks offers a rich set of features that cater to every stage of the data processing pipeline. Some of the significant functionalities include:
- Collaborative Workspace: This feature allows data scientists and engineers to work together in real-time using notebooks. The ability to share insights and iterate on data projects enhances productivity.
- Unified Data Analytics: Databricks simplifies the process of data ingestion from various sources. This integration allows businesses to analyze data in a unified manner, making decision-making more straightforward.
- Scalability: The platform provides elasticity in its computing resources. Users can easily scale up or down based on workload requirements.
- AI and Machine Learning: Databricks includes built-in support for various machine learning frameworks. This helps teams leverage advanced analytics and predictive modeling without complex setup processes.
These functionalities benefit users by providing an environment where they can handle large volumes of data efficiently. They reduce the need for extensive coding while enhancing analytical capabilities, thus saving time and resources.
Unique Selling Points
What makes Databricks stand out among its competitors? Several aspects are particularly noteworthy:
- Integration with Cloud Providers: Databricks runs on popular cloud platforms like Amazon Web Services and Microsoft Azure. This flexibility allows users to choose the environment that best suits their infrastructure needs.
- Delta Lake: This feature enhances data reliability by providing ACID transactions on data lakes. It ensures data consistency and allows for easier data operations.
- High Performance: Performance optimization capabilities allow businesses to run their jobs faster, significantly reducing data processing times.
- Innovation Focus: Continuous updates and new functionalities show Databricks' commitment to innovation. Staying ahead with frequent enhancements is a strong point for many organizations.
Intro to Databricks SKU
Databricks SKU is an essential topic for businesses looking to optimize their data management and analytics processes. Understanding the various SKUs available can significantly impact a company’s ability to handle vast amounts of data efficiently. The Databricks platform unifies data analysis and machine learning, making it invaluable for organizations aiming to derive actionable insights from their data.
What is Databricks?
Databricks is a cloud-based platform designed to facilitate big data analytics and machine learning. It integrates seamlessly with Apache Spark, which allows users to process data at scale. This means businesses can run complex queries and models without the need for extensive infrastructure. The platform offers a collaborative workspace where data engineers and scientists can work together. It provides tools suited for both light data exploration and heavy production workloads.
Moreover, Databricks automates cluster management, optimizing resource utilization, which helps in lowering computing costs. Users can benefit from features such as rapid data ingestion, streamlined workflow management, and built-in security.
Defining SKU in the Context of Databricks
In the context of Databricks, SKU refers to the different plans and pricing options available for businesses. Each SKU caters to specific business needs, whether they focus on data engineering, data science, or machine learning. By defining SKUs, Databricks enables businesses to make informed choices based on their operational demands and performance requirements.
Choosing the right SKU is not only about price; it encompasses features, capabilities, and the level of support provided. The complexity of SKUs can be daunting but understanding them is crucial for maximizing investment. Different SKUs can reflect varying capacities, additional features like security enhancements, and the number of users supported.
"Understanding the right SKU can mean the difference between a successful deployment and wasted resources."
This understanding helps in aligning the technical capabilities of Databricks with a company's strategic goals. Organizations can effectively plan their data initiatives and budget accordingly.
The Role of Databricks in Data Management
In modern business environments, effective data management is crucial for decision-making and operational efficiency. Databricks plays a pivotal role in data management by providing a unified platform. This platform enables organizations to process large amounts of data efficiently, implement machine learning algorithms, and analyze data insights in real time.
Databricks simplifies the complexities that come with handling diverse data sources and types. It allows companies to focus more on extracting value from their data rather than managing underlying infrastructure. The integrated workspace facilitates collaboration among data scientists, engineers, and analysts. By fostering this collaboration, businesses can leverage a more streamlined approach to data-driven solutions, ultimately leading to better outcomes.
Cloud-Based Data Solutions
Databricks operates entirely in the cloud, which brings several advantages to businesses. One significant benefit is the scalability it offers. Organizations can easily adjust their compute resources according to workload demands. This dynamic allocation of resources allows firms to handle spikes in data processing needs without the immediate need for on-premise hardware upgrades.
Another advantage of cloud-based solutions is accessibility. Users can access Databricks from anywhere with an internet connection. This facilitates remote work, which is increasingly important in today's work environment.
The infrastructure also ensures that data is automatically backed up and secure, reducing the risks associated with data loss. Companies can take advantage of the advanced security measures integrated into cloud services. This helps in compliance with regulations such as GDPR and HIPAA, which are essential for businesses handling sensitive information.
- Scalability: Adjust compute resources based on demand.
- Accessibility: Work from anywhere with internet access.
- Security: Benefits from advanced cloud security features.
Integration with Apache Spark
At the core of Databricks’ functionality is its seamless integration with Apache Spark. This powerful framework enables big data processing and analytics. Databricks leverages Apache Spark’s capabilities, allowing users to execute large-scale data processing tasks in parallel. This results in faster data processing times, which is essential for real-time analytics.
Using Spark through Databricks provides a simplified experience. Users can utilize Apache Spark’s capabilities without deep technical expertise. The graphical user interface makes it accessible for users at various skill levels. This accessibility is especially valuable for small to medium-sized businesses that may not have a fully equipped data science team.
Moreover, the integration supports various programming languages, including Python, R, and Scala. This flexibility allows data professionals to work within their preferred environment, optimizing productivity.
"Databricks' integration with Apache Spark enables organizations to empower their data teams with faster, more efficient processing capabilities."
Types of Databricks SKUs
Understanding the types of Databricks SKUs is crucial for businesses looking to leverage the full potential of this platform. Each SKU is tailored to different use cases and levels of complexity, catering to various business needs. This section explores the Standard SKU, Premium SKU, and Enterprise SKU, highlighting their specific features, benefits, and considerations.
Standard SKU
The Standard SKU is designed for small to medium-sized businesses, especially those starting with data analytics and management. This package provides essential features that allow teams to create, manage, and analyze data seamlessly.
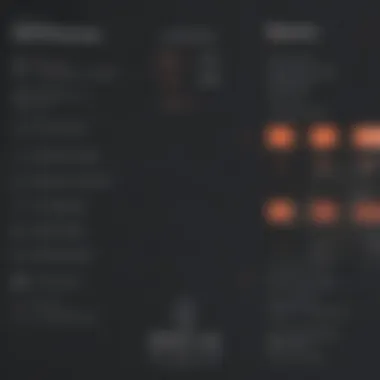
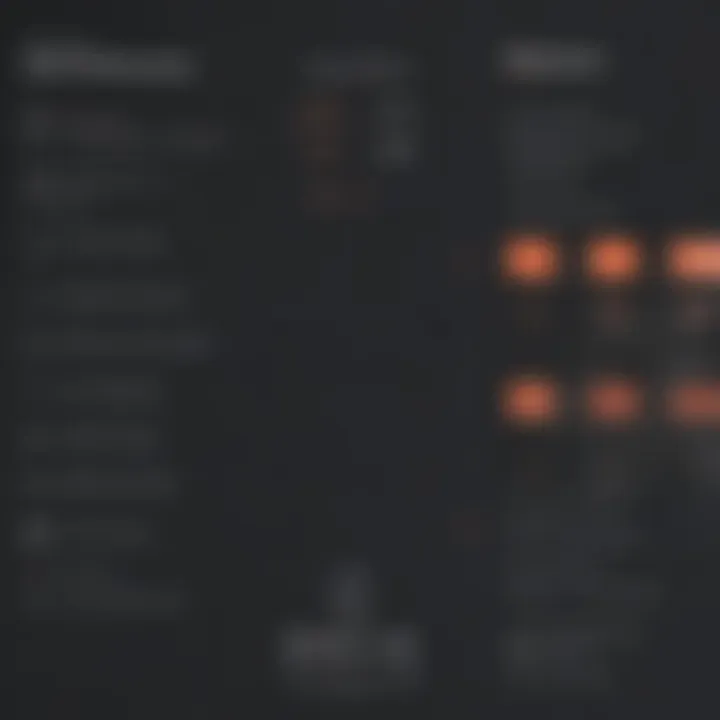
Key characteristics include:
- Cost-Effective: The Standard SKU is often more affordable than its counterparts, making it accessible for organizations with budget constraints.
- Core Features: It includes essential functionalities like collaborative notebooks, data integration, and robust security measures.
- Scalability: Users can begin with initial small datasets and expand as needed, paving the way for growth in data operations.
However, it is important to recognize that while the Standard SKU is an excellent starting point, it may not cater to the needs of organizations requiring high-level performance or extensive features. This SKU primarily suits companies focused on essential data analytics without complex requirements.
Premium SKU
The Premium SKU offers advanced capabilities suited for companies with more intensive data requirements. It enhances functionalities found in the Standard SKU while integrating additional tools for better performance and collaboration.
Notable features of the Premium SKU include:
- Enhanced Security: Businesses benefit from advanced security features, including role-based access controls, to ensure data integrity.
- Advanced Analytics: This SKU supports more complex analytics scenarios. Ideal for teams requiring sophisticated data modeling.
- Collaboration Tools: Premium SKU users enjoy advanced collaborative functionalities, fostering teamwork across departments.
This SKU is suitable for businesses that are transitioning to larger data projects. As they grow, so do their data needs, making the Premium SKU an essential investment for those looking to enhance their data capabilities while maintaining security and collaboration.
Enterprise SKU
The Enterprise SKU targets large organizations or those with substantial data processing needs. This package provides a comprehensive set of features designed to handle extensive data workloads and offers a more tailored experience.
Key components of the Enterprise SKU are:
- Custom Solutions: Organizations can utilize custom solutions, ensuring the SKU meets specific operational needs.
- Full-Scale Integration: The Enterprise SKU excels at integrating with existing systems, making it suitable for complex infrastructures.
- Dedicated Support: Users receive access to dedicated support teams, which is critical for maintaining operations in high-demand environments.
While this SKU comes at a higher price point, the return on investment can be significant for enterprises looking to fully optimize their data architecture. Understanding the capabilities of the Enterprise SKU allows organizations to make informed decisions about resource allocation for data initiatives.
Evaluating Databricks SKU Offerings
Evaluating the offerings of Databricks SKUs is crucial for businesses looking to harness the full potential of their data. Understanding the variety of SKUs available allows for informed decision-making aligned with organizational needs. Effective evaluation can help identify the right tools that provide the best scalability, performance, and cost-effectiveness. Key elements include the specific use cases that each SKU addresses, as well as their unique benefits for different business contexts.
Use Cases and Applications
Databricks SKUs serve multiple purposes across various sectors. Each SKU can be more suitable depending on a business's specific objectives and operational scope.
- Standard SKU: This is often chosen by smaller companies or teams. It is ideal for those who need basic data engineering and analytics functionalities without extensive customization. Applications typically involve straightforward analytics and data integration tasks.
- Premium SKU: Larger organizations with multi-faceted operations may benefit from this SKU. It supports advanced analytics and features like collaborative notebooks, sophisticated machine learning capabilities, and optimized performance. Typical use cases include predictive analytics and large-scale data transformations.
- Enterprise SKU: For businesses needing extensive governance and security, the Enterprise SKU includes features like role-based access control and enhanced support. Suitable for companies with complex data workflows where compliance and data protection are paramount. Applications here can include extensive data warehousing and sophisticated reporting.
The practical application of each SKU will depend heavily on the specifics of the business context and its data requirements, ensuring that the selection aligns with strategic goals.
Comparative Benefits of Each SKU
Choosing the right SKU not only impacts functionality but also affects performance and cost. Here, we outline comparative benefits:
- Standard SKU:
- Premium SKU:
- Enterprise SKU:
- Simplicity in deployment and usage
- Lower cost, ideal for startups
- Sufficient for basic analytics needs
- Enhanced collaboration tools
- Advanced analytics capabilities
- Suitable for teams needing interactivity in projects
- Extensive support and customization options
- Stronger data governance features
- Tailored solutions for compliance requirements
Choosing the correct SKU ensures that a business can optimize its workflow and yield better insights from its data. The evaluation process can lower risks associated with misaligned resources and increase overall efficiency.
By understanding the distinct use cases and benefits, businesses can strategically position themselves to maximize their data investments and enhance their competitive edge.
Pricing Structure of Databricks SKUs
The pricing structure of Databricks SKUs is a crucial aspect for businesses considering integration of the platform into their operations. As organizations navigate data management solutions, understanding this structure helps in making informed decisions tailored to their specific needs. Pricing plays a pivotal role not only in budget allocation but also in evaluating the potential return on investment. By assessing the pricing model, businesses can gauge their operational efficiency and the effectiveness of their data strategies.
Understanding Databricks Pricing
Databricks employs a multifaceted pricing strategy that can accommodate various business requirements. The pricing is determined based on multiple factors including compute resources and the level of support desired. Businesses can choose options that allow flexibility and scalability, adapting to their changing data environments. Understanding these components ensures that companies select the best value for their investment while managing costs effectively. Rigorous consideration of the pricing strategy can optimize expenditure without compromising quality of service.
Pricing Models for Businesses
Databricks offers two main pricing models: consumption-based pricing and flat rate pricing. Each has distinct characteristics that cater to different business objectives.
Consumption-based Pricing
Consumption-based pricing aligns costs directly with the usage of resources. This model allows businesses to pay only for what they consume, making it particularly appealing for organizations with fluctuating workloads. A key characteristic of this model is its adaptability, allowing businesses to scale up or down based on actual data needs. This approach is beneficial because it preserves budget control while maximizing resource utilization.
The unique feature of consumption-based pricing is its granular billing method. While it can lead to cost savings, it may also lead to unpredictable expenses, especially during peak usage times. Companies must track their usage closely to avoid exceeding budget expectations.
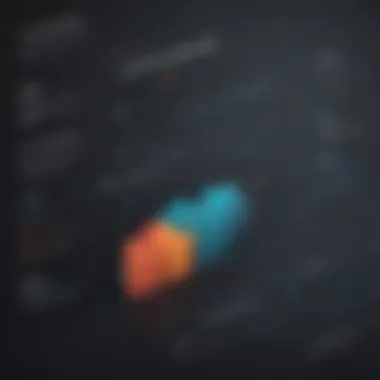
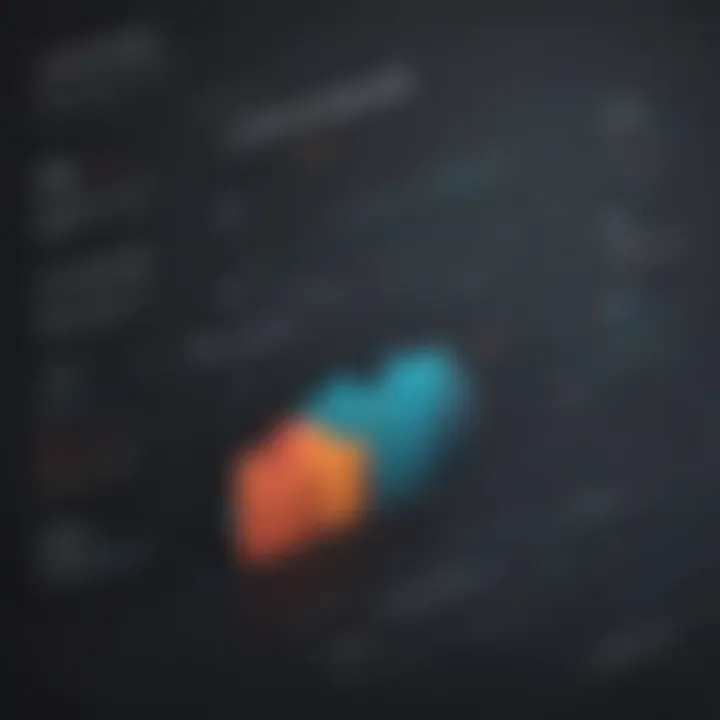
Flat Rate Pricing
Flat rate pricing offers a straightforward alternative where businesses pay a fixed cost regardless of resource usage. This pricing model simplifies budgeting, allowing for easier projections of costs over time. It is popular among organizations seeking predictability in expenses.
A unique feature of flat rate pricing is its stability, making it easier for businesses to allocate budget without worrying about sudden spikes in costs. However, the downside lies in the potential for overpayment if usage remains consistently low. Organizations may not fully utilize the resources they pay for, leading to possible inefficiencies.
In summary, understanding the pricing structure of Databricks SKUs involves a careful analysis of consumption-based and flat rate pricing. Each model presents different advantages and challenges. Businesses need to consider their data usage patterns and budget constraints to choose the option that best aligns with their strategic goals.
"Choosing the right pricing model can significantly influence the success of your data initiatives."
By thoroughly analyzing these options, businesses can maximize their investment in Databricks, fostering an environment for robust data engineering and analytics.
Implementation Considerations
Understanding the implementation considerations of Databricks SKU is crucial for businesses aiming to optimize their data strategy. The effectiveness of any software, including Databricks, heavily relies on how well it aligns with the specific needs and goals of the organization. Here's an exploration of important factors to keep on mind when implementing Databricks SKU.
Assessing Your Business Needs
Every organization has distinct needs shaped by its size, industry, and specific objectives. Assessing these needs is the first step in a successful adoption of Databricks SKU.
- Identify Data Use Cases: Understanding what data you have and how you plan to use it is essential. Are you looking to analyze historical data for insights, or do you want to process real-time streaming data? Each scenario may require different capabilities from Databricks.
- Evaluate Existing Infrastructure: Assess your current data architecture and infrastructure. Compatibility with Databricks can significantly impact performance and efficiency.
- Consider Team Skill Set: Evaluate the existing skills of your team. If your team is already proficient with Apache Spark, the integration will be smoother, thus enhancing the overall effectiveness of Databricks.
- Budget Constraints: Budgeting is vital. Understand the financial impact of implementing Databricks SKUs—both initial costs and recurring expenses.
This assessment allows businesses to tailor their approach to effectively utilize the features of Databricks.
Choosing the Right SKU
The choice of the appropriate SKU is a pivotal step in leveraging Databricks for your business. Each SKU offers different features and capacities, allowing organizations to select based on their requirements.
- Standard SKU: Suitable for smaller teams or businesses that require basic capabilities without extensive features. Ideal for testing and development environments.
- Premium SKU: This SKU accommodates advanced analytics and larger workloads, best for medium-sized businesses intending to scale their data services.
- Enterprise SKU: Tailored for large enterprises requiring comprehensive features, high scalability, and robust security options. This is crucial for organizations where data compliance and governance are top priorities.
In summary, businesses must carefully analyze their needs and the available SKUs. The right decision can lead to better data management, cost efficiencies, and enhanced analytics capabilities.
Choosing the right SKU is not just a technical decision; it is a key strategic move that can influence the future of your data operations.
Deployment Options with Databricks
The deployment options available with Databricks play a crucial role in determining how effectively businesses can utilize the platform. These options not only provide flexibility in terms of infrastructure but also align the Databricks usage with the specific needs of various organizations. Understanding these deployment options is essential for businesses seeking to optimize their data management and analytics strategies.
Cloud Deployment Versus On-Premises
Cloud deployment is often the preferred choice for many businesses. It leverages the scalability and performance of cloud environments, allowing companies to quickly adjust resources based on their requirements. For example, businesses can scale their data processing capabilities up or down seamlessly, which is particularly beneficial for handling fluctuating workloads. Additionally, cloud deployment often reduces the overhead and complexity associated with managing physical hardware.
On the other hand, on-premises deployment can be advantageous for businesses with strict compliance requirements or those that need direct control over their data assets. Although this option may incur higher upfront costs and longer deployment times, it allows organizations to maintain their data within their own secure infrastructure. This control can be critical for industries that handle sensitive information, where data residency and security are paramount.
"Choosing between cloud and on-premises deployment often hinges on specific business needs and regulatory requirements."
Both deployment methods come with trade-offs. The selection depends on factors such as organizational size, industry regulations, and existing IT infrastructure. Hence, businesses must evaluate their specific circumstances to make an informed decision.
Hybrid Deployment Models
Hybrid deployment models offer an attractive middle ground between cloud and on-premises options. This model allows organizations to take advantage of both environments, enabling them to optimize performance, ensure data security, and manage costs effectively. Businesses can store sensitive data on-premises while leveraging cloud resources for broader analytics purposes.
One common scenario involves handling large volumes of data processing in the cloud while keeping critical data secure on internal servers. This flexibility can lead to improved agility, allowing businesses to rapidly respond to market changes while safeguarding sensitive information.
Implementing a hybrid model can be complex, requiring robust orchestration and data management strategies. Organizations must ensure seamless integration between the cloud environment and their on-premises infrastructure. Proper planning and ongoing management are essential.
In summary, the choice of deployment option significantly impacts how businesses utilize Databricks. Whether opting for cloud, on-premises, or a hybrid approach, organizations should assess their own needs, capabilities, and objectives to select the most effective deployment strategy.
Impact of Databricks SKU on Data Engineering
The advent of Databricks SKU has notably transformed the landscape of data engineering. For small to medium-sized businesses, the focus is often on optimizing data processes and facilitating seamless collaboration among teams. Understanding the impact of Databricks SKU in these areas can lead to substantial operational improvements and strategic advancements.
Streamlining Data Processes
Streamlining data processes is one of the most significant benefits attributed to Databricks SKU. Traditional data engineering workflows can be cumbersome, often involving multiple tools and platforms that do not communicate well with each other. Databricks addresses this problem by offering a unified platform where data engineering, data science, and analytics coexist. This integration reduces the need for data engineers to juggle between different tools, which can lead to inefficiencies.
With Databricks, tasks like data ingestion, transformation, and storage can be performed in a single environment. This can enhance speed and accuracy, making data available in near real time for analysts and decision-makers.
Additionally, the platform provides robust support for Apache Spark, enabling scalable data processing. The built-in collaborative notebooks allow data engineers and data scientists to work together more effectively, minimizing the usual silos between teams. This collaboration not only boosts productivity but also fosters innovation by allowing for quicker iterations and experimentation with data.
Enhancing Data Collaboration
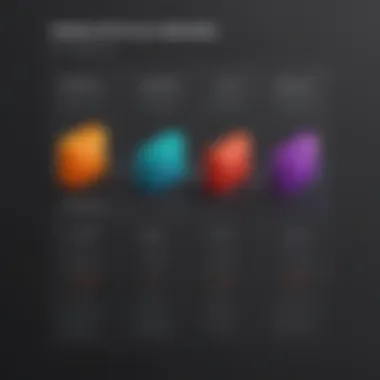
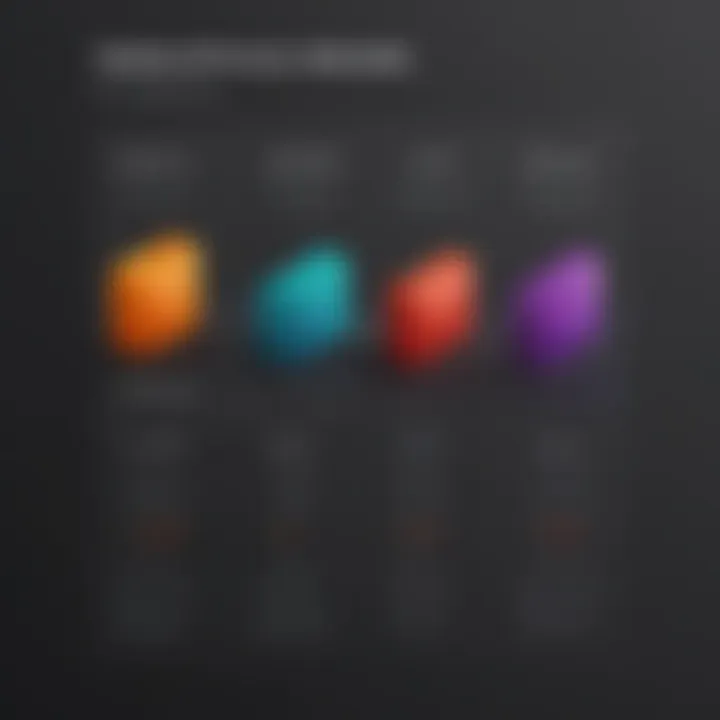
Enhancing data collaboration is another key impact of Databricks SKU on data engineering. In many organizations, data silos can impede a company's ability to become data-driven. Databricks fosters a culture of collaboration by making data accessible across various teams, whether they are in data engineering, business intelligence, or other areas.
The collaborative workspaces and notebooks allow multiple users to engage with data projects simultaneously. This means that stakeholders from different departments can contribute insights and make informed decisions together.
Moreover, the integration with various business intelligence tools enhances data accessibility. Users can easily visualize and analyze data within the Databricks environment. By breaking down barriers, teams can discuss findings, share feedback, and drive actionable insights effectively.
When businesses leverage Databricks SKU for collaboration, they not only enhance productivity but also improve the overall quality of data-driven decisions.
"Collaboration in data engineering leads to better insights and enhances overall project outcomes."
Databricks SKU and Business Intelligence
The intersection of Databricks SKU and business intelligence is a critical area that organizations must navigate effectively. As data continues to grow exponentially, businesses are increasingly relying on advanced analytics to drive decision-making. Databricks provides the tools necessary to transform raw data into actionable insights, enabling organizations to remain competitive in their industries.
One of the primary benefits of utilizing Databricks SKUs is their ability to simplify complex data processing workflows. Companies can build and deploy data-driven applications with increased efficiency. This not only reduces time spent on data preparation but also enhances the reliability of the analytics outputs. By streamlining these processes, businesses can focus more on strategic initiatives and less on technical hurdles.
Leveraging Data for Informed Decisions
Companies need to leverage data effectively for informed decisions. Databricks facilitates this by offering a unified analytics platform that integrates various data sources. This centralization is crucial, as it allows businesses to analyze patterns in real-time. For example, retail businesses can monitor customer behaviors and adjust their strategies accordingly.
Utilizing Databricks SKUs empowers organizations to:
- Access real-time data: Real-time processing enables timely responses to market changes.
- Analyze large datasets: Businesses can handle big data without sacrificing performance.
- Enhance predictive capabilities: Analytical models can be developed to forecast trends, improving decision-making processes.
"The right data paired with robust analytics leads businesses to smarter decisions."
These advantages collectively enhance an organization's ability to make strategic decisions based on solid data foundations.
Data Visualization Tools in Databricks
Effective visualization is crucial in business intelligence. Databricks SKU offers various data visualization tools that can help transform complex data sets into understandable visual formats. Such tools are instrumental in identifying trends, patterns, and outliers within data.
The visualization capabilities in Databricks allow teams to:
- Create interactive dashboards: Users can design dashboards that update in real-time based on data changes.
- Leverage built-in libraries: Utilizing libraries like Matplotlib and Plotly makes it easier to visualize data creatively.
- Share insights seamlessly: Collaboration is enhanced as teams can share visual reports and dashboards across departments.
In summary, integrating Databricks into business intelligence strategies not only improves data handling but also enhances storytelling through data visualization. This dual capability is essential for modern businesses looking to thrive in a data-centric landscape.
Best Practices for Maximizing Databricks Investment
To fully leverage the power of Databricks SKU for your business, there are several best practices that can guide planning and execution. These practices can help ensure that investments made into this data platform are effective and yield significant returns. Understanding how to properly implement and utilize Databricks requires careful consideration of strategies relating to staff training and ongoing performance assessments.
Training and Onboarding Staff
One of the cornerstone practices in maximizing any investment in Databricks is ensuring that staff are adequately trained. It is crucial for all users—from data engineers to analysts—to understand the capabilities of Databricks and how it integrates with existing workflows.
Training typically involves a combination of formal education, workshops, and hands-on sessions. Here are some key points to consider:
- Customized Learning Paths: Tailor training programs to specific roles within the organization to make the learning process more relevant.
- Encourage Continuous Learning: The technology landscape can shift quickly, so provide opportunities for ongoing education through courses and certifications related to Databricks.
- Utilize Community Resources: Engage with forums like Reddit or resources at Wikipedia to encourage knowledge sharing and best practices among staff.
By investing in high-quality training, businesses can empower employees to utilize Databricks to its fullest potential, leading to increased productivity and innovation.
Regular Performance Assessments
Regular performance assessments are another vital practice that businesses should adopt. Monitoring how effectively Databricks is used can reveal areas for improvement and ensure alignment with business objectives. Here are aspects to focus on during these assessments:
- Review Key Metrics: Establish clear KPIs to measure the performance of data processes through Databricks.
- Solicit Feedback: Regularly gather insights from team members on their experiences with the platform. This can help identify obstacles or challenges they face.
- Adjust Strategies as Needed: Use data gathered from assessments to make informed decisions about further investments in training, resources, or tool integration.
"Regularly assessing performance allows for more agile responses to challenges and ensures that Databricks continues to meet business needs effectively."
In summary, applying best practices in training and assessment cultivates a workforce that is not only skilled but also adaptable. This adaptability is essential in optimizing the return on investment in Databricks and achieving business goals.
Epilogue
The conclusion serves as an essential part of any comprehensive guide, bringing together the multifaceted aspects discussed throughout the article. For this article, it emphasizes the significance of understanding Databricks SKU in today's business environment. Leveraging data effectively can provide a competitive edge. Businesses of all sizes, especially small and medium-sized enterprises, can benefit from a well-structured approach to their data management and analysis needs. Databricks SKU offers various tiers that cater to specific requirements, hence addressing the diverse needs of organizations.
Summary of Key Insights
This article has highlighted several critical insights into Databricks SKU. The key takeaways include:
- Flexibility in Offerings: Databricks provides a range of SKUs including Standard, Premium, and Enterprise, each tailored to different business requirements.
- Cost-Effectiveness: Understanding the pricing models can facilitate more informed financial decisions, optimizing the return on investment.
- Deployment Versatility: Whether opting for cloud deployment or hybrid models, businesses can choose what best suits their operational strategies.
- Enhanced Data Collaboration: Databricks fosters better data sharing and team collaboration, which are vital components for effective data-driven decision-making.
- Future Growth Potential: Continuous advancements in features and capabilities signify that Databricks will remain a significant player in the data analytics landscape.
Future Perspectives on Databricks SKU
The future of Databricks SKU appears promising, driven by ongoing innovations in technology and shifting business needs. Key future perspectives include:
- Increased Integration with AI and ML: As more businesses adopt artificial intelligence and machine learning, Databricks is likely to expand its offerings to support these technologies, enhancing predictive analytics capabilities.
- Scalability Features: As organizations grow, their data needs also expand. Future updates may introduce features that facilitate seamless scaling, allowing businesses to adapt swiftly.
- Enhanced User Experience: Expect a push towards simplifying user interfaces and processes, making it easier for businesses to utilize the full potential of Databricks SKU.
- Community and Collaboration: Increased collaboration within the user community can lead to shared best practices, enhancing overall data strategies.
Ultimately, understanding Databricks SKU goes beyond mere comprehension; it is about leveraging the tools to propel business success in a data-centric world. The insights presented in this article aim to guide business owners and IT decision-makers towards informed choices that align with their strategic objectives.